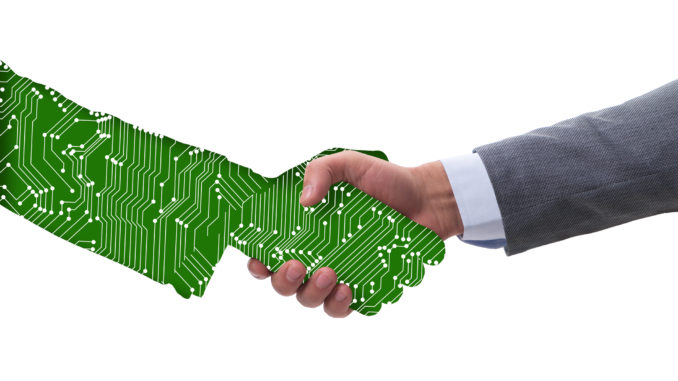
(Editor’s Note: What follows is Part 2 of a 3-part article that will be shared in the pages of A&G Magazine each Monday through July 1. The abstract for the 3-part series appeared here. The author, Lisa A. Pratico, was profiled here in the spring of 2023.)
It cannot be denied that AI can be an effective tool for combating climate change, but its role in contributing to carbon emissions cannot be ignored.
My next statement may be controversial, but I believe we are at cross-roads of inflection where we all to focus on responsible consumption of AI and whether it is really needed for all the uses to which we are applying it. After all, you don’t need a hammer to fix every problem in your house.
But how bad is the problem?
According to an article published in Nature.com, the growing push to quickly harness AI to speed up and scale efforts and find solutions to common challenges underscores the need to closely examine the technology’s impact on the environment and ethical concerns around transparency and fairness. “The launch of the AI Innovation Grand Challenge at the 2023 United Nations climate summit was a significant step in the push for AI in climate action in developing countries. The Grand Challenge was focused on how to achieve the Sustainable Development Goals — the world’s blueprint to end hunger and poverty, clean up the environment, and provide health care for all by 2030.”1 The article discussed the potential for exponential growth in daily usage, which should cause one to pause and ensure AI is being applied to the right situations and problems, not to all situations. According to the article, if generative AI was used daily by billions worldwide, the total annual carbon footprint may reach about 47 million tons of carbon dioxide, contributing to a 0.12% increase in global CO2 emissions.
According to the authors’ analysis, a GenAI chatbot application that assists 50 call center workers, each supporting four customers per hour, can generate around 2,000
1 Indevir Singh Banipal, Sourav Mazumder, “How to make AI sustainable”, Nature.com, February 29, 2024. https://www.nature.com/articles/d44151-024-00024-8
tonnes of carbon dioxide annually. Water consumption from large-scale adoption of GenAI — half of the world population sending 24 queries a day/per person — may match the annual fluid intake of more than 328 million adults. Moreover, data centers serving AI technology workloads host large-scale computing infrastructures, especially arrays of graphic processing units. This infrastructure generates high heat energy when they serve the AI workloads, which must then be removed from the data center server room to avoid overheating and keep the machines running within the operating temperature zone. Two types of cooling systems are typically used: cooling towers and outside air cooling. Both require water.
According to research the computing power needed for AI to work – especially LLMs – requires huge amounts of energy, which can have a significant effect on the environment. The scope and size of the energy usage, however, depends on what phase of the ML lifecycle you measure – training or deployment. Research posted by HollisticAI (which is a product company: www.hollisticAI.com) as well as several research programs and universities assessing AI’s impact on the environment have noted:
- LLMs are notably energy-intensive compared to other AI systems due to the high amount of compute needed for these models to work.
- From the manufacturing of chips to the powering and cooling of data centers, LLMs use huge amounts of energy at every phase in their lifecycle.
- While the training phase of an LLM is typically seen as the most energy- intensive, inference poses a potentially higher environmental cost.
One research article put it best, the impact of AI on the environment is the subject of discourse, with arguments for both positive and negative effects. There is a fine line between AI for good and AI for environmental degradation. Today, companies want to seize the benefits of AI, which distinctively involves reducing the company’s carbon footprint. However, AI’s carbon emissions differ as per the techniques involved in training it. A coin always has two sides, but are business and IT leaders aware of the pros and cons to the two sides? It cannot be denied that AI can be an effective tool for combating climate change, but its role in contributing to carbon emissions cannot be ignored either.2
According to a research article by Niklas Sundberg, “Tackling AI’s Climate Change Problem,”3 AI has a fast-growing carbon footprint, stemming from its voracious appetite for energy and the carbon cost of manufacturing the hardware it uses. Since 2023, the most extensive AI training runs have been using exponentially more computing power, doubling every 3.4 months, on average.4 According to Sundberg, several factors contribute to the carbon footprint of AI systems including:
- Data centers and transmission networks account for 1% to 1.5% of global electricity use and 0.6% of global carbon emissions, requiring significant reductions to achieve net-zero emissions by 2050.
- The electronic waste generated by information technology, including AI systems, is substantial, reaching 57 million tons annually, with potential environmental repercussions equivalent to the Great Wall of China’s weight.
- Factors contributing to the carbon footprint of AI systems include the energy intensive training of LLMs, data storage and processing requirements, energy sources, water consumption in data centers, and the production and disposal of AI hardware. OpenAI’s ChatGPT-3 LLM is made possible by its 175 billion- parameter model, one of the largest when it was launched. Its training alone is estimated to have used 1.3 gigawatt-hours of energy (equivalent to 120 average U.S. households’ yearly consumption) and generated 552 tons in carbon emissions (equivalent to the yearly emissions of 120 U.S. cars).5 OpenAI’s latest model, GPT-4, is rumored to be 10 times larger.6
- The carbon intensity of energy sources powering AI systems significantly impacts their carbon footprint, with renewable energy sources offering potential reductions.
- Water consumption in data centers is substantial, with AI development contributing to increased water use, posing challenges for companies with ambitious environmental targets. Microsoft revealed in its most recent environmental report that its global water use increased 34% from 2021 to 2022 (to approximately 1.7 billion gallons, or more than 2,500 Olympic-sized swimming pools). Google reported a 20% increase in water use during the same period, an increase that outside experts have linked to its AI development.7
- The production and disposal of AI hardware contribute to carbon emissions and the growing e-waste problem, emphasizing the importance of recycling and resource recovery to build a sustainable economy. The global volume of electronic waste is predicted to reach 120 million tons annually by 2050, double what it is today. The material value of the same e-waste — only 20% of which gets formally recycled — is approximately $62.5 billion.
Despite significant environmental costs, AI plays a vital role in promoting sustainability and addressing climate change by optimizing renewable energy utilization, improving agricultural practices, enhancing supply chain efficiency, and optimizing data center operations through machine learning algorithms. 3
There is now focus from researchers and organizations who are starting to track and pay attention to the impacts of AI. According to a study in MIT’s Technology Review, AI’s carbon footprint is bigger than you think, big tech companies don’t share the carbon footprint for training and using their massive models, and according to MIT there hasn’t been a standardized way of measuring the emissions for which AI is responsible.8
While those researching this issue now know training AI models is highly polluting, the emissions attributable to using AI have been a missing piece until now. MIT published research that calculated the real carbon footprint of using GenAI models. Generating one image using GenAI uses as much energy as charging your smartphone, according to the study from researchers at the AI startup Hugging Face and Carnegie Mellon University. This has big implications for the planet, because tech companies are integrating these powerful models into everything from online search to email, and they get used billions of times a day.9
If you were ever in technology, you probably have referred to data as oil. When mined and refined, it is a highly valuable and lucrative commodity. Now with all the news and focus on AI, the industry is being compared to oil. However, as MIT stated it seems the metaphor may extend further. The process of deep learning has an outsized environmental impact like its fossil-fuel counterpart. Researchers at the University of Massachusetts Amherst released a report estimating that the amount of power required for training and searching a certain neural network architecture involved the emissions of roughly 626,000 pounds of CO2, equivalent to nearly five times the lifetime emissions of the average U.S. car, including its manufacturing.10 MIT research took this finding further and continued to say the issue gets even more severe in the model deployment phase, where deep neural networks need to be deployed on diverse hardware platforms, each with different properties and computational resources.11
Researchers and organizations are now starting to track and pay attention to the impacts of AI. According to a study in MIT’s Technology Review, big tech companies didn’t share the carbon footprint for training and using their massive models, and according to MIT there wasn’t a standardized way of measuring the emissions AI is responsible for.8 As of March 6, 2024, the securities and exchange commission requires sizable public companies to disclose their emissions.12
What has been done to resolve the problem?
It’s not all bad as researchers and universities focus on the impacts of AI on carbon emissions, things are improving. Artificial intelligence has some major sustainability issues, and several large organizations and universities are tackling these, propelling us into technology advancements in chipsets and neural network designs that not only combat AI carbon emissions but are also advancing technology hardware and software. There is even research data on how AI should leverage clean power grids to combat the AI carbon emissions issue.
Neural Network Advancements: To combat the sustainability issues, MIT researchers developed a new automated AI system for training and running certain neural networks. Results indicated that, by improving the computational efficiency of the system in some key ways, the system can cut down the pounds of carbon emissions involved – in some cases to low triple digits. The researchers invented an AutoML system with improved computational efficiency and a much smaller carbon footprint. The researchers’ system trains one large neural network comprising many pretrained subnetworks of different sizes that can be tailored to diverse hardware platforms without retraining.13,14
Clean Power Grid Use: Vijay Gadepally, a research scientist at the MIT Lincoln lab who did not participate in the original MIT research, had similar thoughts. Knowing the carbon footprint of each use of AI might make people more thoughtful about the way they use these models. As noted in the MIT article, carbon footprints of AI in places where power grids are relatively clean, such as France, will be much lower than it is in places with a grid that is heavily reliant on fossil fuels, such as some parts of the US. While the electricity consumed by running AI models is fixed, Gadepally believes we might be able to reduce the overall carbon footprint of these models by running them in areas where the power grid consists of more renewable sources. While climate change is extremely anxiety inducing, it’s vital we better understand the technology sector’s effect on our planet. Studies like Gadepally’s might help us come up with creative solutions that allow us to reap the benefits of AI while minimizing the harm. 8
According to Sundberg, best practices for sustainable AI can be more broadly expressed as the three R’s: Relocate, Rightsize, and Re-architect.
- Relocate:TransitioningAIoperationstoareaswithaccesstorenewableenergy sources like solar or wind power can significantly reduce carbon emissions. Placing computing workloads in regions with high renewable energy availability, such as Quebec, Canada, can lead to substantial emissions reductions, up to sixteen-fold compared to the US average. Cloud-based computing, especially when using data centers powered by renewable energy, offers additional emissions savings compared to on-premises setups.
- Rightsize:OptimizingAImodelsandapplicationsbyrightsizingcomputingand storage resources can substantially decrease carbon footprints. Companies can improve performance and energy efficiency by using processors and systems specifically designed for machine learning tasks. Additionally, strategies like limiting GPU power draw and time-shifting demanding workloads to times of lower carbon intensity contribute to energy savings.
- Rearchitect:DesigningrobustsoftwareandhardwarearchitecturesforAI models is essential for scalability and maintaining low-latency responses while minimizing energy consumption. Choosing efficient machine learning model architectures, such as sparse models, can significantly reduce computation without sacrificing quality. Managing technical debt post-deployment is crucial to avoid performance issues and technology risks, highlighting the importance of ongoing optimization and redesign efforts to enhance efficiency and quality.
AI’s contributions to solving the climate crisis can outweigh its negative climate impacts, but only if the AI industry adopts practices that emphasize ESG sustainability, makes sustainability central to its AI ethics guidelines, and actively seeks opportunities to reduce the environmental footprint of AI technologies. Users of AI must also be aware of the factors that contribute to the environmental impacts of these tools to help guide their own use of AI and add sustainability to the list of criteria they use to evaluate AI vendors.3
In part 3 of this article, we will look at why Enterprise Architecture must be engaged to ensure AI is being designed and deployed appropriately within the business to ensure maximum value with the proper techniques and deployment.
1 Indevir Singh Banipal, Sourav Mazumder, “How to make AI sustainable”, Nature.com, February 29, 2024. https://www.nature.com/articles/d44151-
2 L Gaur, A Afaq, GK Arora, N Khan, “Artificial intelligence for carbon emissions using system of systems theory,” Ecological Informatics – Elsevier, 2023. https://www.sciencedirect.com/topics/earth-andplanetary- sciences/carbon-dioxide-emission
3 Niklas Sundberg, “Tackling AI’s Climate Change Problem”, MIT-Sloan Management Review, Dec 12, 2023,
4 “AI and Compute,” OpenAI, May 16, 2018, https://openai.com
5 Patterson, “Carbon Emissions and Large Neural Network Training.”
6 M. Schreiner, “GPT-4 Architecture, Datasets, Costs and More Leaked,” The Decoder, July 11, 2023, https://the-decoder.com.
7 M. O’Brien and H. Fingerhut, “Artificial Intelligence Technology Behind ChatGPT Was Built in Iowa — With a Lot of Water,” Associated Press, Sept. 9, 2023, https://apnews.com.
8 Melissa Heikkila, “AI’s carbon footprint is bigger than you think,” AI – MIT Technology Review, December 5, 2023, https://www.technologyreview.com/2023/12/05/1084417/ais-carbon-footprint-is-bigger-than-youthink/
9 Melissa Heikkila, “Making an image with generative AI uses as much energy as charging your phone,” AI – MIT Technology Review, December 1, 2023, https://www.technologyreview.com/2023/12/01/1084189/makingan- image-with-generative-ai-uses-as-much-energy-as-charging-your- phone/?truid=&utm_source=the_algorithm&utm_medium=email&utm_campaign=the_algorithm.unpaid.eng agement&utm_content=12-04-2023
10 Emma Strubell, Ananya Ganesh, Andrew McCallum, “Energy and Policy Considerations for Deep Learning in NLP,” College of Information and Computer Science – University of Massachusetts, Amherst, June 5, 2019. https://arxiv.org/pdf/1906.02243.pdf
11 Karen Hao, “Training a single AI model can emit as much carbon as 5 cars in their lifetimes,” AI- MIT Technology Review, June 6, 2019, https://www.technologyreview.com/2019/06/06/239031/training-a-singleai- model-can-emit-as-much-carbon-as-five-cars-in-their-lifetimes/
12 Evan Bush, “U.S. companies will have to start telling the public about their climate risks,” NBC-Climate in crisis, March 7, 2024, https://www.nbcnews.com/science/environment/us-companies-will-start-tellingpublic- climate-risks-rcna142105
13 Rob Matheson, “Reducing the carbon footprint of artificial intelligence,” MIT News Office, April 23, 2020, https://news.mit.edu/2020/artificial-intelligence-ai-carbon-footprint-
14 #:~:text=MIT%20researchers%20have%20developed%20a%20new%20automated%20AI%20system%2 0for,down%20to%20low%20triple%20digits.