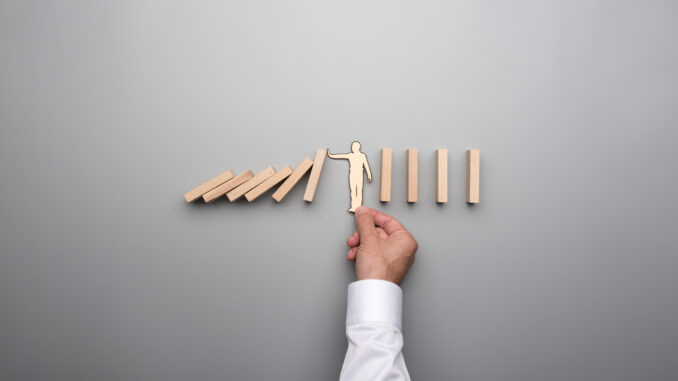
By Dr. Gopala Krishna Behara
AI Technology is changing the way the world does business. Artificial Intelligence (AI) refers to the use of AI to create new content, like text, images, music, audio, and videos. It can produce a variety of content covering images, video, music, speech, text, software code and product designs. AI helps in faster product development, enhanced customer experience and improved employee productivity and automate business processes.
According to Gartner, “Any organization in any industry, especially those with very large amounts of data, can use AI for business value.” AI is primed to make an increasingly strong impact on enterprises over the next five years.
By 2025, global AI revenue is projected to reach $118.6 billion, indicating a significant increase from the $9.5 billion generated in 2018 – Tactica
There exist various definitions of AI Governance across the industry. Some of them are,
Schneider defined AI Governance for businesses as “The structure of rules, practices, and processes used to ensure that the organization’s AI technology sustains and extends the organization’s strategies and objectives.”
Butcher and Beridze characterize AI Governance as “A variety of tools, solutions, and levers that influence AI development and applications.”
Winfield and Jirotka defined AI ethical governance as “A set of processes, procedures, cultures and values designed to ensure the highest standards of behavior”.
Lack of AI Governance leads to the following enterprise level issues:
- Siloed and ad-hoc AI software development driven by a tactical approach
- Enterprise cannot maintain proper alignment of business and IT
- Inability to rapidly respond to AI technology changes driven by business/technology challenges and no holistic enterprise AI view
- Data becomes stale or obsolete and hence becomes useless or less useful impairing strategic decision-making based on this stale/obsolete data thereby defeating the very purpose of AI
- Complex, fragile, and costly AI data interfaces between applications and data
- Incomplete future state of AI reference architecture leads to slow and ineffective decision-making
As an Architect, the author sees the current state of AI Governance adoption/implementation by the Industry/Enterprises are ad hoc and unorganized. Also, there is a lack of understanding of the state of AI governance within the enterprise governance structure.
According to the author, AI governance is defined as “A system of standards, guidelines, rules, practices, processes, and tools based on AI technologies aligns with the Enterprise’s strategies, objectives, and values.”
AI Governance is the responsibility of not only the IT executives (CXO and IT Directors) but also executives from Business organization as well, along with critical participation from Enterprise Architects, Domain Architects, Subject Matter Experts, and other support staff throughout the enterprise. Successful AI enabled enterprise designing is not simply a function of the IT organization but needs total Enterprise participation.
This whitepaper covers AI Governance and its alignment with Enterprise Governance, Challenges in AI Governance, Guiding principles of AI Governance, AI Governance Framework, and benefits of AI.
ALIGNMENT WITH ENTERPRISE GOVERNANCE
The various governance systems across the enterprise and their alignment with the AI Governance are briefly described in this section. AI Governance does not exist in Isolation, it should be part of overall Governance system of the entire enterprise.
Corporate Governance is an overarching governance structure of an entire enterprise. It is a management body that manages Enterprise Operations in relation to its internal and external stakeholders. This body acts like an Approval authority and decision-making body. At the corporate level, AI Governance aligns with the enterprise objectives, rules, policies, and decision making.
EA Governance consists of Enterprise Leadership, Organizational structure, direction, and processes that ensure Information Technology (IT) sustains and extends the enterprise’s vision, mission, strategies, and goals in a planned manner. AI Governance leverages the guidelines, principles, standards, reference architectures defined at the enterprise level. In summary, AI Governance is in alignment with the EA Governance.
IT Governance executes corporate governance. It is defined as the processes that ensure the effective and efficient use of IT in enabling an enterprise to achieve its goals. AI functionalities are implemented using software code that is modeled as algorithms. These algorithms are standalone applications or integrated with the enterprise information systems to provide additional capabilities and better performance and automation. AI Governance enables IT principles, IT architecture, IT infrastructure and business needs across enterprise. AI governance at the enterprise level can be viewed as a subset of IT governance.
The following diagram depicts the indicative end to end governance of an Enterprise and their relationships. It depicts AI Governance and its alignment with Enterprise Governance Structures.
Fig 1: AI Governance & its alignment with Enterprise Governance Structures
AI Governance is defined as a system of rules, practices, processes, and AI tools that are adopted to ensure enterprise use of AI solutions that aligns with the business strategies, objectives, and values.
Data Governance helps in managing the data across the enterprise. Helps in defining the AI algorithms and AI systems in processing the data. Data Governance team provides strategic direction, guidelines, policies, standards, and usage of procedures. It also helps in measuring and monitoring the effectiveness of data governance on a continuous basis. AI algorithms and AI systems process data. The business functionalities, outputs, and value depend on the data.
AI Governance overlaps with Data Governance. AI Governance is the subset of IT Governance. IT Governance and EA Governance overlaps each other. IT Governance is the subset of Corporate Governance.
CHALLENGES IN AI GOVERNANCE ADOPTION
The main challenges faced by the enterprises today in implementing AI solutions are,
Data Preparation: Identification of data sources for AI, labeling of data for algorithms, data management, data governance, data policies, data security, and data store are the challenges for the enterprises.
Data Quality: Poor data can create biases in the AI system. Data quality can impact AI model capabilities and performance.
Reliability: Trained models are black boxes and has no clue to end user. This may lead to false, harmful, and unsafe results.
Security Risks: Cloud models may leak proprietary data, IP, PII, and model interaction history.
Technology complexity: Data preparation for LLMs, algorithm design, building of models, training the models is a complex task. Compute identification for training, cloud identification and deployment are complex tasks.
Huge Customization: Enterprise business needs require extensive Fine tuning of base foundation models and prompt engineering.
Skill Gap: AI initiatives require Machine Learning/Deep Learning/Prompt Engineering/Large Language Model expertise to build and train Foundation Models. Many enterprises lack these skilled resources and are not available in-house. Enterprises building algorithms and models to meet the business requirement will be a challenge.
GUIDING PRINCIPLES OF AI GOVERNANCE
The Objectives of the AI Governance are:
- Ensure enterprise is adopted pre-trained foundation models and complied
- Guide the decision-making process to maintain AI Solution coherence
- Maintain the relevancy of the enterprise to meet changing requirements
Guiding Principles of AI Governance are:
- Accountable: Enterprise Users should be accountable for AI systems. Assess the impact of the system on employees and enterprises.
- Transparency: AI Systems should be transparent and understandable and allow users for easy decision making.
- Open: Build AI Open Systems, sharing source code, training data, and other relevant information, all while protecting personal information, system integration.
- Fairness: AI systems should treat all users fairly. AI systems are designed to provide a similar quality of service for identified demographic groups.
- Inclusive: AI systems should empower everyone and engage people. AI systems are designed to be inclusive in accordance with enterprise accessibility standards.
- Security: Provide enterprise data governance and security. AI platforms must provide robust encryption, multi-level user access authentication, and authorization controls.
- Governance: AI governance to be developed end to end, Governance, ethics, integrity, and security need to be built in from inception. Develop AI systems interact with entire enterprise providing integrity from the foundation level. Empower the individuals. Establish the process of continuous human learning and improved decision making.
- Skill Management: provide training so that employees developing and using AI solutions have the responsible design, function, and implementation skills needed to make AI-based services better.
AI GOVERNANCE FRAMEWORK
The AI Governance Framework helps Enterprise to Manage, Govern, Monitor, and Adopt AI activities, practices, and systems across enterprise.
AI Governance Framework defines a set of metrics that can be used to measure the success of the framework implementation.
The AI Governance Framework comprises the following key elements required to govern, monitor, and adopt AI practices and systems.
- AI Governance Organization Structure
- AI Governance Operating Model
- AI Taxonomy
- AI Tools & Technologies
- AI Metrics
- AI Monitoring
The Enterprise AI Governance Framework is depicted below.
Fig 2: AI Governance Framework
AI Governance ORGANIZATION STRUCTURE
Establish an executive team for identifying and overseeing the AI initiatives across the enterprise. Define a clear vision and strategy for AI implementation aligned with the enterprise goals and business functions. Develop practical communications to, and appropriate access for employees.
Setup AI Governance across enterprise. Define roles and responsibilities of individuals involved in AI development, deployment and monitoring. Foster the collaboration between AI experts, domain experts and business stakeholders. Establish a centralized, cross-functional team to review and update AI governance practices as technology, regulations, and enterprise needs. The AI Organization Structure consists of:
- AI Governance Office
- AI Center Of Excellence
- AI & Data Science Teams
The AI Governance Office is accountable for ensuring the AI is responsible, ethical, transparent, fair, and trustworthy. The AI Governance Office need to focus on:
- Strategize the Business
- Business Problem and Business Opportunity addressing with AI
- Establish AI Business Requirements
- Alignment of AI with Organization Goals
- Define Business Value and its measurement criteria
- Set and Lead Governance
- Establish an AI Governance Office to help guide enterprise decisions
- Ensure that AI strategy to align with business strategy
- Clear communication of objectives of AI to respective stakeholders
- Obtain peer Buy-in
- Articulate the benefits of executing the AI, as well as the costs and risks to the business
- Define Metrics
- Access to and active participation of all the stakeholders
- Bring in the business
- Establish a culture of responsible AI
- Maintain Momentum
- Monitor the AI initiatives through regularly scheduled reviews
- Demand regular updates on modernization projects
- AI adoption as an ongoing process requiring regular evaluation
- Resolve any AI related issues
- Encourage employee interest in AI
AI Center Of Excellence oversights the AI insights. It focuses on,
- Conduct regular AI adoption reviews
- Deploy experienced team of consultants with right mix of skills
- Identify the applications that quality for the adoption of AI in terms of meeting business needs in a cost-effective and reliable manner
- Incorporate auditing. This help businesses develop and deploy policies to protect the business from risks such as copyright infringement and proprietary data leakages
- Build a business case
- Define Business Processes that impacted by AI
- Articulate the costs and risks of each potential AI project, including the opportunity cost of doing nothing
- Democratize ideas, limit production. Prevent employees from launching untested and unregulated AI projects
- Allow employees to experiment without the ability to operationalize the use of AI
- Determine a recommended course of action
- Create an AI adoption framework
- Development of AI/ML standards, policies, and procedures
- Development of AI architecture
- Standardize AI architecture, tools, and technologies, including ML operations (MLOps) tools, across the enterprise
- Alignment on tools and technologies used by AI/data science teams
- Streamline data sources, talent, and technology
- Establish Centre of Excellence
- Upskill employees in AI
- Building use cases and minimum viable products
- Prompt definition and fine tuning them
AI Team to focus on
- Collect relevant and meaningful data
- Availability and time commitment from IT stakeholders and key SMEs/resources for information sharing, workshops, interviews, surveys, validation of findings, and related activities as per schedule
- Ask right set of questions very specific to customer pain areas leading AI exercise
- Identify Dynamic data
- Check for existing data and use appropriately
- Prepare dynamic data. Dynamic data includes tables, images, videos, text, code etc
- Review data sets for compliance with risk, privacy, ethics, and legal requirements
- Prompt identification
- Identification of Prompts
- Adjust the prompts AI uses in the initial stages
- Fine tune the prompts to address inaccurate and biased outputs
- Build Target Architecture
- Create target reference architectures
- Design AI/ML solutions
- Design, implement, and deploy the AI solutions
- Create AI adoption Roadmap
AI OPERATING MODEL
The AI governance operating model defines the design and operational implementation of a governance structure that delivers measurable business value to the enterprise while managing model lifecycle and risks and ensuring trustworthy and ethical AI.
AI TAXONOMY
AI Taxonomy defines AI Approach, AI Governance Processes, AI policies, procedures, and standards. It also covers Data Governance and data security. Establishes the Risk Management Framework, and the use of AI across Enterprise.
Specific definitions for key concepts and terms are listed below.
AI Approach is a way in which a task, activity, or process is executed and in which the performance is measured to meet the criteria covering principles, policies, systems, processes, approaches, and techniques.
Blueprints –
- Plan or guide, commonly used in construction, laid out logically and including essential elements to address and follow as building progress
- A blueprint is a plan or design document AI reference architecture of enterprise. It covers,
- Large Foundation Models, trained on huge quantities of unstructured and unlabeled data to perform specific tasks. It acts like a platform for other models.
- Prompt Engineering, process of designing, refining, and optimizing input prompts to guide a generative AI model toward producing desired outputs.
- Embeddings, ML technique that allows for semantic understanding of data
Guiding Principles – Principles are general rules and guidelines, intended to be enduring and seldom amended, that inform and support the way in which an enterprise sets about fulfilling its mission.
AI Policies – It covers Policies and procedures to support implementation of AI governance. It covers, Use of Data for AI/ML initiatives and AI Impact Assessment Policy.
AI Methodologies – A methodology represents a package of practical ideas and proven practices for a given area of activity, such as the planning, design development or management of IT-based systems.
AI Governance Processes – Process is sequence of operations or events, possibly taking up time, space, expertise, or other resource, which produces specific outcome. The AI Governance process covers Data Usage Process, Data Review Process, Data Approval Process. It also covers AI Model definition process, Model Review Process and Model approval process. Governance processes also address the monitoring of the adoption of the AI Policies and Standards.
AI Standards – A set of criteria, voluntary guidelines, and best practices. The AI guidelines and standards are:
- Create an oversight so that humans can be accountable and in contact. Assess the impact of the system on people and enterprises.
- Design AI systems to intelligently for the decision making. AI systems are designed to inform people that they are interacting with an AI system.
- AI systems are designed to provide a similar quality of service for identified demographic groups.
- AI systems are designed to be inclusive in accordance with enterprise accessibility standards.
- AI platform must provide a comprehensive catalog of AI-based software services across enterprises.
- Train data be available for the enterprise AI systems.
- AI platform must provide robust encryption, multi-level user access authentication, and authorization controls.
- AI platform must support multi-cloud operation. Generative AI platforms must be optimized to take advantage of differentiated services.
- Develop AI systems interact with entire enterprise providing integrity from the foundation level. Empower the individuals. Establish the process of continuous human learning and improved decision making.
AI Risk and Compliance – AI Governance needs to address the alignment with enterprise risk management and ensuring compliance with regulations and assessment frameworks. AI Risk management should audit the AI Tools usage and effective usage of AI Models across enterprise.
AI TOOLS AND TECHNOLOGIES
Evaluate AI tools for the enterprise business. The tool needs to adhere to the enterprise AI standards like security, privacy, data handling and compliance. The tool needs to empower the stakeholders to deliver business needs and continuously improve the experiences it generates against business metrics. The AI Tool should consist of the following capabilities,
- Data analysis and data visualization
- Tools for creating AI reports and dashboards
- Data quality, Data cataloging
- AI Model management, including capturing model metadata and model versioning
- AIOps, MLOps technologies, tools, and platforms to monitor model performance in production, visualize metrics, and capture model performance data
- Role-based access to the models and dataset
AI METRICS
To ensure that AI is progressing well, and adding value to the enterprise, there is a need to define metrics for measuring effectiveness of it. However, AI metrics is still an emerging field and there are no defined standards. In addition, current literature does not contain a tool to measure the success of AI implementation.
AI Metrics not only helps in gauging the long-term impact of AI, but also helps in measuring the true value of AI which will enable the Enterprise to plan more effectively and efficiently.
Measuring an AI metrics is necessary to:
- Determine how effectively and efficiently the process or service satisfies the customer
- Improved ability to seize new business opportunities
- Make decisions based on FACT and DATA
- Improved agility
- Ability to extend systems to meet increasing demand
- Better ability to outsource
Measurement should:
- Translate customer expectations into goals
- Evaluate the quality of processes
- Track improvements
- Support enterprise strategies
AI MONITORING
AI tools provide support for strategic decision making by capturing vital enterprise context, along with content development and analysis capabilities across the business, information, technology and solution architectures.
It helps the stakeholders to analyze and optimize the portfolio of business strategies, enterprise Al structures, business processes / tasks and activities, information flows, applications, and technology infrastructure.
AI GOVERNANCE BENEFITS
Significant cost savings are achieved with right AI governance model across enterprise, in addition to the following benefits:
- Enhance Customer Experience
- Improve Operational Efficiencies
- Automate Business Processes
- Identify new customer journeys and identify new audiences
- Enhance creativity and the ability to use create tools
- Transform campaigns, audiences, experiences, journeys and insights
- Create ideas for new Products
- Reinvent workflows
CONCLUSION
In Summary, AI Governance is an integrated approach, a series of processes, a cultural orientation, and a set of owned responsibilities that ensure the integrity and effectiveness of the enterprise AI adoption. It enables management, monitoring, and control of all AI activities within an enterprise.
AI Governance overlaps with Data Governance. AI Governance is the subset of IT Governance. IT Governance and EA Governance overlap each other. IT Governance is the subset of Corporate Governance.
AI Governance is the responsibility of not only the IT executives (CXO and IT Directors) but also executives from Business enterprise as well, along with critical participation from Enterprise Architects, Domain Architects, Subject Matter Experts, and other support staff throughout the enterprise.
AI Governance Office is responsible for the overall AI vision, strategy, architecture, solution alignment, and AI architecture implementation governance for all IT projects. It is the primary oversight body for Enterprise that has delegated authority from the AI strategy group to make most Enterprise decisions.
Acknowledgements
The author would like to thank Santosh Shinde of BTIS, Enterprise Architecture division of HCL Technologies Ltd for giving the required time and support in many ways in bringing this article as part of Architecture Practice efforts.
About Author
Dr. Gopala Krishna Behara is an Enterprise Architect in BTIS Enterprise Architecture division of HCL Technologies Ltd, India. He has a total of 28 years of IT experience. Reached at gopalakrishna.behara@hcl.com.
Linked In: https://www.linkedin.com/in/gopalbehara/
Disclaimer
The views expressed in this article/presentation are that of authors and HCL does not subscribe to the substance, veracity, or truthfulness of the said opinion.