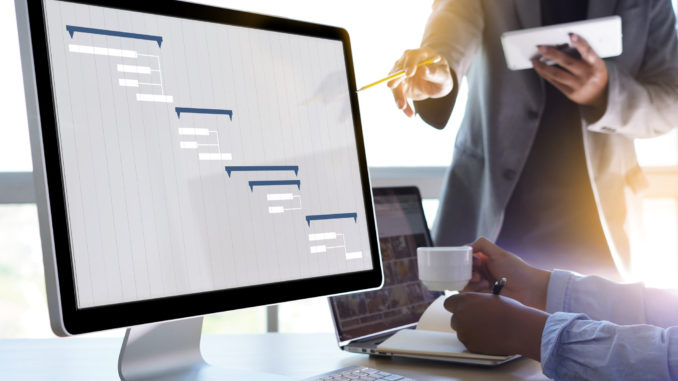
In an age where computers live alongside humankind, the question of whether a project needs a manager is legitimate. Autonomous software systems make daily life-and-death decisions – access systems, vehicles, aircraft, medical, and warfare systems may soon act on inputs without the intervention of human decision-making or validation (Munir, 2019). Software architects continuously seek ways in which autonomous systems may find and eliminate human bias, thus allowing decisions based on the data, using sound decision processes independent of the effect of human bias (Sulaimon, Ghoneim, & Alrashoud, 2019). Moreover, humans tend to trust robots much the same way they trust humans (Howard & Borenstein, 2019). Thus, the question – are projects better without the effect of human bias?
The use of analogous and parametric planning – using patterns and statistical projections from previous projects – is a standard practice in the project profession. Additionally, project managers (PM) engage automated systems for modeling projected outcomes based on data collected over time, giving the PM the ability to project the probability of positive outcomes based on proposed scenarios. Furthermore, since the late 1980s, PMs have explored artificial intelligence in planning and controls. With the advancements in artificial intelligence (AI) decision-making, the software can mimic a PM’s decision process to enhance resource leveling, risk management, and intelligent scheduling processes (Bhavsar, Shah, & Gopalan, 2019; Munir, 2019).
AI software largely works by spotting patterns in data sets. Rather than traditional programming, some AI systems use data to train and validate algorithms. For example, autonomous vehicle systems use data gathered through replays of live traffic patterns, vehicle data collection using sensors collected in live driving, and sophisticated integration of decision algorithms (Markkula, Romano, Madigan, Fox, Giles, & Merat, 2018). Such training of software systems may enhance the ability to audit and control business processes (Bhavsar, Shah, & Gopalan, 2019).
Perhaps, similar to AI, and more specifically Machine Learning, human decision making requires the detection, sampling, and selection of inputs over time – through experience – until a point at which one may choose a best alternative based on the repetition of inputs and outcomes (Huk, Katz, & Yates, 2014; Carr, Jansen, Wimmer, Fu, & Topcu, 2018). However, due to the human condition of stress – including passion and fear – additional inputs to the decision process may at times make one’s decision less than optimal (Paletta, Pszeida, Nauschnegg, Haspl, & Marton, 2019). Although, some researchers agree that in the process of data retrieval, stressors encoded at the time of the event can enhance the ability to recall information (Schwabe, 2017).
However, is an impassionate, unbiased decision the preferential outcome? More recently, project professionals are beginning to see the value in human passion as an input to the project decision matrix. For example, many speakers at the Harrisburg University of Science and Technology’s Project Management Innovation Conference this past June discussed at length the need for attendance to the human condition in project planning and decision-making. Human input in the decision process, even while using AI, allows for expert knowledge and experience to enhance a probable outcome (Cai, Reif, Hegde, Hipp, Kim, Smilkov, … & Terry, 2019).
When interacting with robots, perhaps due to a lack of appreciation for the inherent risk involved in decisions made in the systems development process, stakeholders often do not understand the inherent risk in robot decisions (Howard, & Borenstein, 2019). In addition, Howard and Borenstein point to a potential of culturally specific bias interjected in the systems decision-making process that may not effectively align with the operational environment (2019). Humans, it seems, are still necessary to balance the raw inputs experienced by even the most advanced Deep Learning computer system processing (Bond, Mulvenna, Wan, Finlay, Wong, Koene, … & Adel, 2019).
More importantly, when giving strategic decision-making authority to robotic systems, command and control is lost, and ethical and moral issues arise (Roff, 2014). Roff argues that human intervention is necessary to ensure the correct translation of tactical actions from strategic objectives in a shifting environment (2014). Strategic decisions are often the outcome of a series of incremental decisions that require practical analysis of the present context (Bateman & Zeithaml, 1989). Therefore, it is likely the human bias – conformance to cultural and organizational norms – that protects the strategic objectives of the project and, ultimately, the program.
Unlike AI systems, a PM’s primary role in every project is an active bias to fulfilling the overarching organizational strategy by protecting the scope, schedule, and cost of the assigned engagement. An AIs analytic process has relative value in application to alignment with strategic objectives that can only be assessed and resolved by the PM. Additionally, there are qualitative and subjective business reasons behind a PM’s decision process that an AI is yet unable to mimic. Therefore, do not set aside your project leaders just yet.
Bibliography
Bateman, T. S., & Zeithaml, C. P. (1989). The psychological context of strategic decisions: A model and convergent experimental findings. Strategic management journal, 10(1), 59-74.
Bhavsar, K., Shah, V., & Gopalan, S. (2019). Business Process Reengineering: A Scope of Automation in Software Project Management Using Artificial Intelligence. International Journal of Engineering and Advanced Technology (IJEAT), 9(2), 3589-3595.
Bond, R. R., Mulvenna, M. D., Wan, H., Finlay, D. D., Wong, A., Koene, A., … & Adel, T. (2019, August). Human Centered Artificial Intelligence: Weaving UX into Algorithmic Decision Making. In RoCHI (pp. 2-9).
Cai, C. J., Reif, E., Hegde, N., Hipp, J., Kim, B., Smilkov, D., … & Terry, M. (2019, May). Human-centered tools for coping with imperfect algorithms during medical decision-making. In Proceedings of the 2019 CHI Conference on Human Factors in Computing Systems (pp. 1-14).
Carr, S., Jansen, N., Wimmer, R., Fu, J., & Topcu, U. (2018, June). Human-in-the-loop synthesis for partially observable Markov decision processes. In 2018 Annual American Control Conference (ACC) (pp. 762-769). IEEE.
Howard, A., & Borenstein, J. (2019). Trust and Bias in Robots: These elements of artificial intelligence present ethical challenges, which scientists are trying to solve. American Scientist, 107(2), 86-90.
Huk A.C., Katz L.N., Yates J.L. (2014) Accumulation of Evidence in Decision Making. In: Jaeger D., Jung R. (eds) Encyclopedia of Computational Neuroscience. Springer, New York, NY. https://doi.org/10.1007/978-1-4614-7320-6_309-2.
Markkula, G., Romano, R., Madigan, R., Fox, C. W., Giles, O. T., & Merat, N. (2018). Models of human decision-making as tools for estimating and optimizing impacts of vehicle automation. Transportation research record, 2672(37), 153-163.
Munir, M. (2019). How Artificial Intelligence can help Project Managers. Global Journal of Management And Business Research.
Paletta, L., Pszeida, M., Nauschnegg, B., Haspl, T., & Marton, R. (2019, July). Stress measurement in multi-tasking decision processes using executive functions analysis. In International Conference on Applied Human Factors and Ergonomics (pp. 344-356). Springer, Cham.
Roff, H. M. (2014). The strategic robot problem: Lethal autonomous weapons in war. Journal of Military Ethics, 13(3), 211-227.
Sulaimon, I. A., Ghoneim, A., & Alrashoud, M. (2019, April). A New Reinforcement Learning-Based Framework for Unbiased Autonomous Software Systems. In 2019 8th International Conference on Modeling Simulation and Applied Optimization (ICMSAO) (pp. 1-6). IEEE.
Schwabe, L. (2017). Memory under stress: from single systems to network changes. European Journal of Neuroscience, 45(4), 478-489.