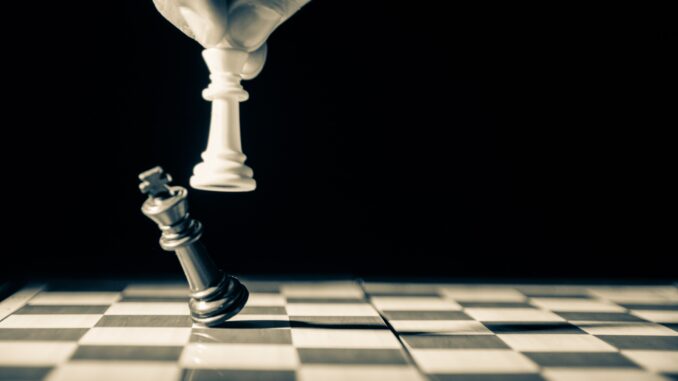
Over the past week a group of thought leaders in which I participate was invited to contribute to an online discussion about the topic of Domain Driven Design and its relationship to business architecture and business capability modeling. Rather than going into the details of the actual topic discussed, I thought it might be interesting to share my experience using AI tools to generate consensus on how to describe architectural concepts.
In an environment with lots of smart, quick-thinking people it can be a challenge to ensure everyone is heard, especially when the primary mode of interaction is videoconferencing. The online format (a Microsoft Teams group chat) gave people time to contribute their thoughts over a period of days rather than minutes.
At various points in the online conversation, participants extracted content from the online discussion board and fed it to a large language model to compare ideas that were present in the dialogue, or to recast the dialogue in a particular person’s voice.
The combination of human interaction with human to machine interaction was fascinating to me as a sociologist. As I participated and reflected upon the conversation that transpired, I observed three patterns that emerged from the process, where the AI tools added value, including:
- All of Us is Better than One of Us,
- Distinguishing Disagreement from “Vehement Agreement,” and
- Making Common Patterns Visible.
I also observed a couple of things we as end users must consider as we incorporate AI tools into our work: end user responsibility for accuracy / correctness of the content, and the ethics of intellectual property ownership.
All of Us is Better than One of Us
Each of us brings our experience and history to a conversation. Our experiences often lead us to develop very strongly held views about our work, the techniques and tools we use, and our identification with a particular role or functional domain. Unfortunately, “architecture” is one of those areas where professionals have strongly held views, to the degree that leaders who have had bad experiences with architecture in general or specific architects tend to discount anything said by someone who has the word “architecture” in their job title.
Some contributors like to think “bottom up”, others “top down.” The “bottom up” thinkers tend to use more words to convey their thoughts, which can result in frustration from the “top down” thinkers who get impatient with having to listen to or read the details. Conversely, the “bottom up” thinkers may become frustrated at the “top down” thinkers’ tendency to over generalize things. Another pattern I see in communication is the distinction high and low sociability, where highly social people want to “talk things out” when lower sociability people want to think by themselves, draw conclusions, and present the conclusions as “finished product.” The presence of these patterns within a group of thought leaders makes it difficult to gain consensus on a topic.
The AI tools used to analyze the dialogue had no knowledge of anyone’s job title or role in the group, they just analyzed the text as if every opinion was equally valid. They made no value judgments about the writing styles of the contributors, nor did they penalize the more “long winded” contributions. The tools summarized the text, highlighted points of agreement as well as disagreement, and even drew diagrams to illustrate some of the concepts presented in the dialogue.
Bottom line: the tools analyzed the text on its own merit, regardless of the characteristics or communications preferences of the authors, which enabled the voices of everyone who contributed to be equally heard, resulting in a final deliverable that was better than any individual’s initial contribution.
Distinguishing Disagreement from “Vehement Agreement”
Reading the text of a week’s worth of dialogue as a back and forth between participants one could get the impression that there was a lot of conflicting opinion about the topic being discussed. However, when the text for participants’ responses to the question, “what is a domain?” was fed to Claude, it created a set of points of agreement, areas of tension, and then compared specific examples to a framework that had been proposed by two of the participants. There was much in common across the contributors’ text, along with one major pattern of disagreement.
In its summary of the text, Claude noted that the main area of disagreement across the participants was the degree to which each participant relied on patterns and principles versus practical application – this is the classic inductive vs. deductive reasoning conflict that is often a reflection of one’s sociability. Or, as Claude summarized, “Yes, this appears to be a case of ‘vehement agreement.’”
Making Common Patterns Visible
The AI tools boiled down thousands of words from multiple contributors into sets of bullet points, organized into categories. This allowed the discussion participants to comment on the distilled conversation as opposed to debate the lengthy text postings point by point. The consensus on our thinking about domains led us to consider how they relate to business capabilities, and after additional discussion and distillation with another AI tool, we were able to agree on language that explains how to use domains to operationalize business capabilities into working tested software products.
Caveat Prompter
The benefits of using AI tools are not cost free. It’s important to verify the results of an AI’s synthesis of text because sometimes the AI misinterprets what was written. For example, during our discussion of capabilities and domains, an AI tool interpreted some of my text as stating that the boundaries of a domain are context dependent when in fact, I was making the opposite argument – that a domain must have a consistent definition that is valid across any contexts in which it participates.
Another consideration is the ethics of intellectual property ownership and citation of participants’ contributions. While there are well established rules in academia for citing the work of others in a research article, who “owns” the synthesis of half a dozen participants’ text into a summary document? When is advance consent required to ensure that participants know that their work is going to be summarized by AI and potentially published as someone else’s point of view?
Five Steps to Integrate AI into Group Decision-Making
The next time you are facilitating or participating in a group decision-making process, consider inviting an AI into the discussion. To make the process productive and enjoyable for everyone, include the following steps.
- Establish the desired outcome – is it a decision for internal use, external use, or both? Will it include supporting text or a blog or research article?
- Agree on the rules of engagement – What will participants be expected to contribute? How will their contributions be recognized in the final work product? Who “owns” the final work product, and gets to decide on the “voice” of the work product?
- Establish the discussion venue – It should be a place where people can interact via text, such as a Slack channel or Microsoft Teams group chat.
- Seed the discussion with probing questions – Encourage people to share their perspectives, to write deeply or broadly.
- Iterate – feed content into the AI, have it summarize & synthesize, then share with the group to sharpen the group’s thinking.
Group Decision-Making & Architecture
A key success factor for architecture decision-making is obtaining stakeholder buy in. However, stakeholders often have conflicting positions and sometimes have had negative interactions with architecture departments or specific architects that make them reluctant to support architecture decisions.
Group decision-making techniques, including the use of AI tools to level the playing field and encourage diversity of thought, can be powerful influence tools in an architect’s toolkit. When people see their ideas incorporated directly into a summarized work product and their contributions are cited in the output, they are more likely to consistently support decisions made by the group.