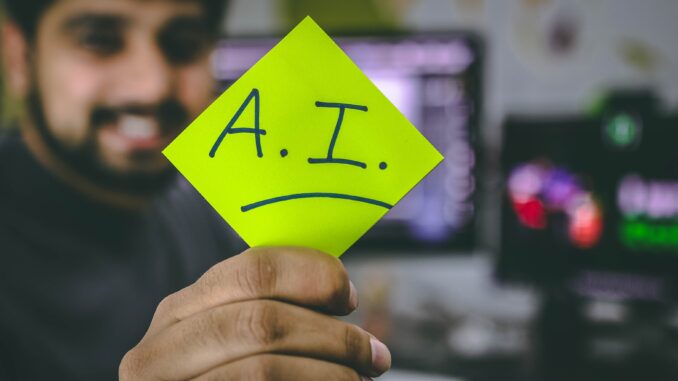
In December, the European Union passed the AI Act, the first major law aiming to regulate technologies that fall under the umbrella of artificial intelligence. The legislation might have arrived sooner, but the sudden success of ChatGPT in late 2022 demanded the act be updated.
The EU’s act, however, does not mention fairness — a measure looking at how well a system avoids discrimination. The field studying fairness in machine learning (a sub-field of AI) is relatively new, so clear regulation is still in development.
Mike Teodorescu, a University of Washington assistant professor in the Information School, recently proposed a new paper suggesting how private enterprise standards for fairer machine learning systems could inform governmental regulation.
The paper was published by the Brookings Institution as part of its series “The Economics and Regulation of Artificial Intelligence and Emerging Technologies.” Christos A. Makridis, a digital fellow at Stanford University, was a co-author.
Teodorescu recently spoke about the paper and the field of machine learning fairness.
Question: To start, could you explain what machine learning fairness is?
Mike Teodorescu: It is essentially concerned with ensuring that a machine learning algorithm is fair to all categories of users. It combines computer science, law, philosophy, information systems and some economics as well.
For example, if you’re trying to create software to automate hiring interviews, you might have a group of HR people interview many candidates with diverse backgrounds and experiences and recommend a binary outcome — hire or don’t hire. Data from actual HR interviews can be used to train and test a machine learning model. At the end of this process, you get accuracy — the percent the model got correct. But this percentage does not capture how well the algorithm performs when considering certain subgroups. U.S. law forbids discrimination based on protected attributes, which include gender, race, age, veteran status and so on.
In the simplest terms, as an example, if you count the number of veterans that you would like to hire, then the algorithm should hire independent of the protected attribute. Of course, this becomes more complex as you have more intersections of subgroups — you might have race, age, socioeconomic status and gender. From a practical perspective, if you have a system of equalities for dozens of values of protected attributes, it is unlikely that all of them will be satisfied at the same time. I don’t think we have a generalizable solution and we do not have yet an optimal way to check for AI fairness.
Question: What is it important for the general public to understand about machine learning fairness?
MT: It helps to understand procedural fairness, which looks at the methods that are used to come up with decisions. A user might want to ask, “Do I know if this software is using machine learning to make some prediction about me? If yes, what kind of inputs is it taking? Can I correct an incorrect prediction? Is there a feedback mechanism by which I can challenge it?”
This principle is actually found in privacy laws in Europe and California, where we can object to certain information being used. That level of transparency would be great in the case of a machine learning algorithm being applied to make some decision about you. Maybe there is an option to select what variables it’s using to show you certain ads. Now, I’m not sure that’s something we will see in the very near future, but it’s something users might care about.
Question: What’s impeding fairness standards from being widely adopted by companies?
MT: I think it’s a problem of incentives. From an economic perspective, companies want to bring products to market as quickly as possible. If users get an app that uses image recognition AI, they likely won’t read the Terms of Service. So they’re probably not going to spend the time to go through training on whether the tool is fair or not. Many users might not even know that it’s possible for a tool to be unfair.
For a company right now, the incentive to develop such systems would be to put the company at the technological forefront and to signal quality — that its AI tools are fairer than its competitors’. But if the users do not know about this being a problem, they may not be worried about which company’s product is fairer. Probably 10 years from now, many more people will care about fairness, just like they do about cybersecurity and data privacy. Cybersecurity wasn’t such a common concern until we had a lot of these breaches.
Question: Would an example of what you’re explaining here be somebody submitting a job application to a company that uses a machine learning algorithm to sort applications? That person wouldn’t necessarily know if there’s a machine learning algorithm sorting these applications, so they certainly wouldn’t know if they’ve been unfairly sifted out.
MT: Precisely, and that concern keeps me up at night. There’s a patchwork of regulations across different countries and states, but there isn’t yet a comprehensive federal regulation about this. There’s a law specifically about automated hiring in New York City. There’s also an EU law that very recently got through, which allows people to contest or determine how their data is being used. There’s a White House set of directives that have been proposed. Eventually, I think there will be a federal law.
Question: Do you see standards arriving first and then driving actual regulation of machine learning fairness?
MT: Yes, regulations are slow. There are a lot of hurdles to pass a law. But standards play more into the economic incentives. There are standards for cybersecurity, quality measurement, WiFi, Bluetooth and so on, but we don’t yet quite have accepted standards for machine learning fairness yet. Usually, an organization produces them. The Institute of Electrical and Electronics Engineers (IEEE) comes up with a lot of technical standards, and actually suggested a few. The standards committees within such organizations usually bring people from industry, academia and government together, and they come up with guidelines that can be updated, so there might be different versions of a standard. That provides a lot more flexibility than regulations. For instance, there are two different quality management manufacturing standards. Most factories have the less strict standard, while the stricter standard for medical manufacturing is very expensive and much more difficult to get. In fairness, you might see a light standard and a much more comprehensive one.
Likewise, standards organizations can have auditing requirements. Once a company complies with a standard, there’s a certain frequency of audits to make sure that the standards continue to be upheld. Having something like that for products that use machine learning would be a great way to improve accountability.