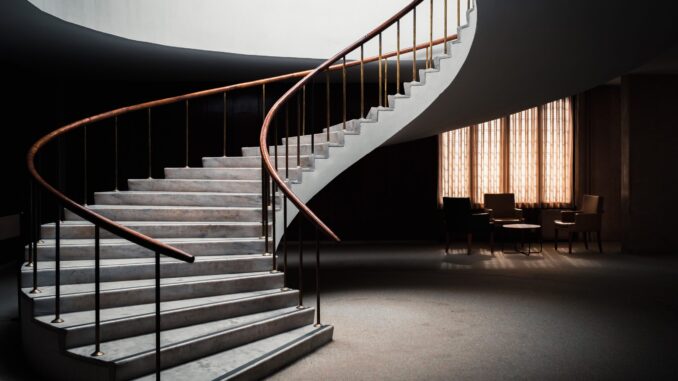
By Jimmy Tam
When I say AI, what comes to mind? For many of us, our first (and perhaps only) experience is using a tool like ChatGPT to answer questions we could easily ask a search engine. Some of us though are starting to use AI for project management and coordination and, in the architectural sphere visualisation tools are becoming more common for client presentations and for a much truer representation of how a new project could really look.
Of course, there is scope for even more value from AI and machine learning. In fact it has the potential to transform the way all of us work in the architecture industry. And with applications that span from generative design software and building information modelling to tools to help with energy efficiency, sustainability and more, the architectural process is set to benefit hugely from the AI revolution.
What’s stopping you?
But there is something getting in the way. AI, usually located centrally, relies on data to perform. Right now much of that data is created and stored across different storage platforms in numerous locations, far from where it needs to be. That alone means you need to consider how to manage your data and how you bring it to your AI model.
For example, transferring data from the edge to a central system can be a slow process. AI tools that rely on data at the edge can be easily disrupted during network outages. And it can be a real effort to keep data synchronised between edge locations and the central system.
It’s a challenge made greater by version control issues which can proliferate when data is stored in different locations.
All these issues mean AI is learning from out-of-date or inaccurate data. The resulting insights will be inaccurate at best, at worst the information produced could mean client relationships are damaged or firms fall foul of regulations.
Get ahead
In my experience, the architecture firms that are already reaping the most benefits from AI are proactive when it comes to managing their data and file systems. They prioritise their data to help their AI learn more effectively and provide better results for their clients. Here are the three steps I suggest to get the most from your AI solutions:
- Make it real-time
For informed decision making, highly accurate modelling and more, make sure you give your AI the most up-to-date information. Identify which data your AI needs to access and provide it in real-time, so that your AI algorithms can analyse data streams as they are generated, provide immediate insights into emerging trends, and respond to changing circumstances or unusual patterns.
A slow and creaky transfer of data from the edge to the centre is not going to give you the results you need. Worse still if you’re relying on an old snapshot from months ago to feed your AI tools. Your insights and outcomes simply won’t reflect what’s happening right now. This could cause real problems for you down the line – inaccurate project assessments, increased costs, even safety risks.
- Think always-on
Imagine, you’re putting together a project proposal. You’re relying on AI insight to help you optimise your designs but an outage has stopped you in your tracks. Without its insight you’re struggling to make sure you’ve fulfilled the brief, that you have allocated the right resources and that your budget projections are accurate. Your deadline is looming.
IT outages can be devastating. They can shut down whole companies and their services. And for AI, if access to data is interrupted, whether that’s through connectivity issues, downtime or datacentre failure, it could dramatically impact results. So prioritise continuous availability to your data, and ensure AI solutions can access critical files and data wherever they’re stored at any time regardless of network disruptions.
- Prioritise version control
Insights from AI are only as accurate as the data it learns from. And if it’s learning from the wrong version of a file, you risk serious errors, which inevitably lead to a lack of trust in AI.
It’s easy to see how it happens. AI using outdated data is likely to generate inaccurate assessments of site progress for example, perhaps miscalculating building dimensions or overlooking recent structural changes. Project managers taking decisions with these flawed insights could go on to allocate the wrong resources or make design modifications that simply won’t work.
So if your data is stored across different systems and locations, identify ways to automate file updates so that changes are reflected immediately wherever they’re stored. And consider how you’ll track changes, manage revisions, and maintain a clear audit trail of data modifications, so that your AI model can identify the most recent version.
I’ll finish with this: by prioritising your data, you can unlock the full potential of AI in your architectural projects. Giving your AI tools access to the right data at the right time, will help you to move beyond the way many of us are still using AI (I don’t need to tell you, it’s so much more than a glorified search engine!) into something more ambitious and transformational.
Tam is the CEO of Peer Software.