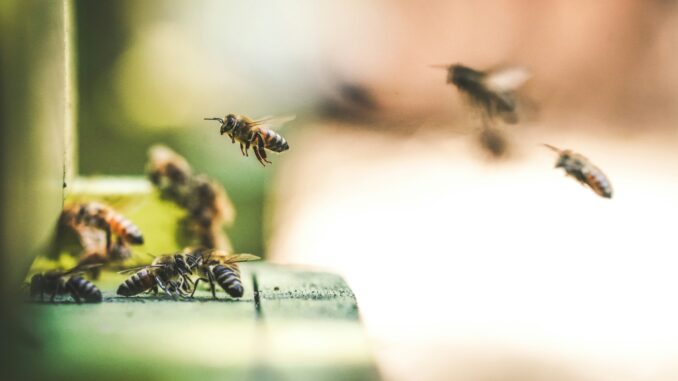
The impact of AI is mind boggling. In just a few years we have gone from AI Assistants to AI Agents, to Agentic AI, where swarms of AI Agents collaborate to meet a common objective. Can you imagine 1000’s of independent AI Agents collaborating and making independent decisions?
Hence the Agentic AI journey is unlike any other. The challenge in automating human intelligence has no precedence. The largest challenge lies in control. We have only designed systems for control. But now we need to architect and design for loss, or lack, of control. Because that is what autonomous means, lack of control.
The challenge for architects is unprecedented. How do we architect for thousands of autonomous Agentic AI agents making real-time decisions in all parts of an organization? It is not a reality yet, but it is coming, and we need to start preparing.
The Agentic Architecture Framework
Agentic AI is likely to create most issues in some areas more than others. The Agentic Architecture Framework identifies seven areas that will require more support in the forms of new or updated frameworks, tools and techniques to support Agentic AI capability-building and architecture development.
Agentic Architecture Framework
Agentic AI Readiness
The journey begins with a current state assessment to gauge organizational readiness. Using a Capability Maturity Model (CMMM) tailored for Agentic AI, organizational capabilities can be assessed across five maturity levels.
The Agentic Readiness Assessment spans ten dimensions that are critical in supporting Agentic AI and ensuring a holistic understanding of readiness to plan and implement AI at scale.
Agentic AI Maturity Dimensions
Agentic AI Strategy
Agentic AI Strategy begins with defining a clear target state across the Agentic AI maturity dimensions and levels. This step establishes the organization’s AI aspirations and provides a benchmark for future transformation. Once the target state is identified, the next step involves conducting a GAP analysis to determine the differences between current capabilities in the previous step, and the organization’s ambition.
With these gaps clarified, organizations can then focus on identifying and quantifying high-impact AI use cases that align with business objectives and support progression toward the target state. These prioritized use cases form the backbone of a strategic roadmap, guiding AI investments and ensuring ongoing and sustainable capability-building and transformation.
Agentic AI Operating Model
The Agentic AI Operating Model defines how AI systems, people, and processes work together to deliver value. It focuses on integrating AI into the organization’s core operations, ensuring that AI agents operate seamlessly within new and existing workflows and alongside human teams.
The operating model encompasses three key components:
- People: The operating model emphasizes the roles and responsibilities of employees in an AI-driven environment. It requires upskilling the workforce to interact with AI agents effectively, and a shift from management and direct control to governance and indirect control.
- Processes: Operational workflows must be redesigned to incorporate AI capabilities and missing workflows will be created. This includes replacing entire systems with swarms of collaborating AI Agents, designing human teams into the process, not the other way around.
- Technology: A robust technological foundation is essential. The model requires scalable infrastructure, secure data pipelines, and API-driven systems to enable autonomous and dynamic interactions by AI agents.
By aligning people, processes, and technology, the Agentic AI Operating Model provides a blueprint for operationalizing AI at scale, ensuring continuous value creation and adaptability.
Scalable Enterprise Architecture
Scalable Enterprise Architecture provides the structural framework for integrating Agentic AI across an organization’s operations. It enables dynamic and autonomous capabilities of AI agents to deliver transformative outcomes by integrating business, data, application, and technology architectures.
- Business Architecture: Aligns AI initiatives with business goals. At higher maturities, AI agents autonomously develop dynamic capabilities to address specific opportunities or issues.
- Data Architecture: Establishes frameworks for managing vast data volumes, including real-time pipelines and secure governance. At higher maturity levels, it enables predictive analytics, empowering AI agents to drive real-time decisions.
- Application Architecture: Enables applications to integrate seamlessly with AI agents. API-driven frameworks support agents in orchestrating workflows, managing online transactions, and delivering real-time insights. At advanced levels, applications enable fully autonomous operations.
- Technology Architecture: Provides infrastructure for AI agents to operate at scale, including cloud platforms, secure servers, and adaptive networks. At higher maturity levels, AI agents optimize resources and infrastructure.
Agentic AI Governance
Agentic AI Governance establishes policies and oversight to manage risks and ensure ethical, secure, and compliant AI operations. Key governance dimensions include:
- Ethical Use and Transparency: Ensuring fairness and trust in AI-driven decisions by implementing explainable AI techniques.
- Risk Management: Proactively addressing biases, security vulnerabilities, and compliance risks through regular audits and testing.
- Regulatory Compliance: Adhering to evolving industry regulations and data privacy laws. This includes implementing mechanisms to track and document AI decisions for regulatory reviews.
- Continuous Monitoring and Accountability: Regular evaluation of AI performance to refine agent behavior and ensure alignment with business needs. Governance frameworks must also include contingency plans for addressing unexpected outcomes.
Governance provides the confidence to scale AI responsibly, balancing innovation with accountability and trust.
Agentic AI Design
Agentic AI Design focuses on how AI agents operate, interact, and deliver value. It encompasses:
- Agent Roles: Defining responsibilities, from automating tasks to complex decision-making. Clear role definitions help prevent overlap and ensure efficient collaboration among agents.
- Interaction Models: Designing human-agent and agent-to-agent interactions via intuitive interfaces and APIs. These models foster seamless communication and enhance user experience.
- Operational Frameworks: Establishing rules for agent behavior, feedback loops, and performance monitoring. This includes developing mechanisms for conflict resolution among agents.
- Agent Types: Classifying agents by function, such as task automation, decision support, or human collaboration. Tailored training data and algorithms are essential for optimizing each agent type.
A key tool in Agentic AI design is the Agentic AI Canvas Agent Canvas, which is designed for organizations aspiring to level 3. It provides a structured approach, starting with a business use case and stakeholders, to define required AI agents, types, roles, interactions, and data flows, including governance to achieve significant functional automation.
The canvas bridges operational alignment and data-driven collaboration, laying the groundwork for scalable and synergistic AI systems.
Agentic AI Canvas
AI Agent Modelling Language
The AI Agent Modeling Language (AIAML) provides a structured framework for defining, designing, and standardizing how AI agents operate and interact within complex enterprise ecosystems. This language enables clear, consistent modeling of agent behaviors, communication protocols, and integration points, ensuring seamless collaboration between AI agents, users, and systems. AIAML serves as the foundation for translating high-level business requirements into operational AI capabilities.
- Agent Definition: AIAML formalizes the characteristics, roles, and responsibilities of AI agents. It captures their decision-making logic, operational boundaries, and objectives, ensuring agents align with business goals.
- Interaction Design: The language specifies how AI agents interact with one another, enterprise applications, and external systems. It includes defining APIs, communication protocols, and data-sharing frameworks to enable real-time, dynamic collaboration.
- Behavioral Modeling: AIAML outlines the decision-making processes and learning mechanisms of agents. This includes specifying rules, algorithms, and feedback loops that guide agent behavior and adaptability.
- Scalability and Optimization: AIAML supports the modeling of resource allocation, scalability requirements, and system optimizations, ensuring agents operate efficiently even under high workloads.
The Agentic Architecture Framework equips architects and organizations with practical tools and roadmaps to adopt Agentic AI. By aligning AI capabilities with strategic goals and embedding ethical practices, it ensures scalable and impactful transitions to autonomous systems.
Jesper Lowgren is an enterprise architect and thought leader specializing in AI Agent and Agentic AI frameworks, tools and techniques. He leads the Enterprise Architecture Microsoft Practice in DXC Technology (Australia), where he develops Agentic AI frameworks and architectures to help customers adopt and scale AI Agents and systems of AI Agents. Jesper is the author of two books on transformation and has a YouTube channel where he shares architecture, AI, and technology thought leadership.