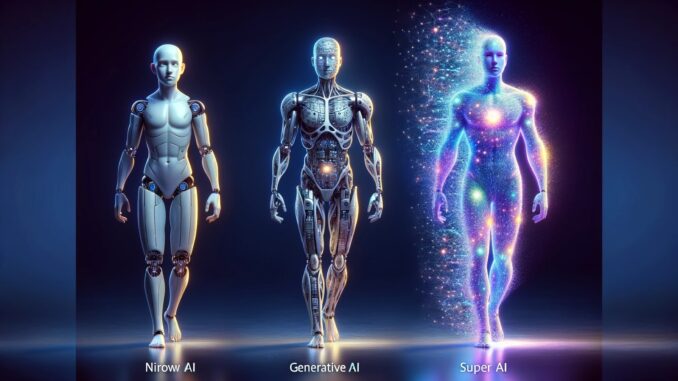
The buzz surrounding artificial intelligence (AI) is deafening, yet a significant disconnect exists between the hype and actual implementation. As an NBC News report highlighted, while AI dominates business conversations, the hype is not translating into real-world use. A survey last November from the Census Bureau in the U.S. found only 4.4% of businesses had used AI recently to produce goods or services. The most recent data from the UK’s Office for National Statistics in 2023 suggest that “when it comes to day-to-day use of AI, 5% of adults have used AI a lot.”
It is still early days in the AI revolution and large language models (LLMs) have captured the lion’s share of attention. However, concerns over the costs and computational demands of LLMs are driving pragmatic executives to explore alternatives. Surprisingly, the development of small language models (SLMs) is outpacing expectations.
Generally speaking, adoption rates are meaningless because the journey towards artificial general intelligence (AGI) systems – encompassing both LLMs and SLMs – requires functionality that has been largely unavailable until now. The insatiable appetite for more data across diverse domains is leading to increased complexity and spiralling costs, often at the expense of performance and reliability.
Vectors: The Catalyst for AI Innovation
Vector databases have recently emerged into the spotlight as the go-to method for capturing the semantic essence of various entities, including text, images, audio, and video content. Encoding this diverse range of data types into a uniform mathematical representation means that we can now quantify semantic similarities by calculating the mathematical distance between these representations. This breakthrough enables “fuzzy” semantic similarity searches across a wide array of content types.
While vector databases aren’t new and won’t resolve all current data challenges, their ability to perform these semantic searches across vast datasets and feed that information to LLMs unlocks previously unattainable functionality.
Here are three key factors driving data growth that enterprise teams must consider in order to maximise the value of their vector solutions:
- Exponential Data Expansion
The ongoing multiplication of data is not a new phenomenon, but we can certainly anticipate a surge driven by the explosion of AI data demands. This trend shows no signs of slowing and will likely accelerate in the coming years.
- Unlocking Unstructured Data
Vectors represent a huge leap in the amount of data that can be processed. By enabling the handling of unstructured data such as images and video, vectors significantly expand the horizons of usable data. Previously, most AI and machine learning (ML) models primarily worked with structured data. Vectors uncover a new frontier of previously untapped data sources.
- Enabling Hyper-Personalised Computing
Real-time data can now be leveraged to provide more specific, hyper-personalised recommendations for users. While aggregated personalisation techniques have been the norm, offering a broad-scale statistical view of collective behaviour, vectors allow for tracking more customised data to feed into AI and ML pipelines. This detailed tracking of each user’s behaviour, both long term and recent behaviour, reveals vast new amounts of data. However, it also introduces additional privacy and security concerns which must be addressed.
Building Blocks for Long-Term AI Innovation
We are in the early stages of leveraging vectors, both in the emerging generative AI space and the classical ML domain. It’s important to recognise that vectors don’t come as an out-of-the-box solution and can’t simply be bolted onto existing AI or ML programs. However, as they become more prevalent and universally adopted, we can expect the development of software layers that will make it easier for less technical teams to apply vector technology effectively.
As organisations embark into their AI-driven journeys, they need to make sure that their data infrastructure can handle the speed, scale, and performance necessary to thrive. By laying the groundwork today with vector technology and acknowledging the scalability it presents, they can position themselves to harness its full potential of vectors and drive meaningful AI innovation in the years to come.
Naren Narendran is the Chief Scientist at Aerospike.