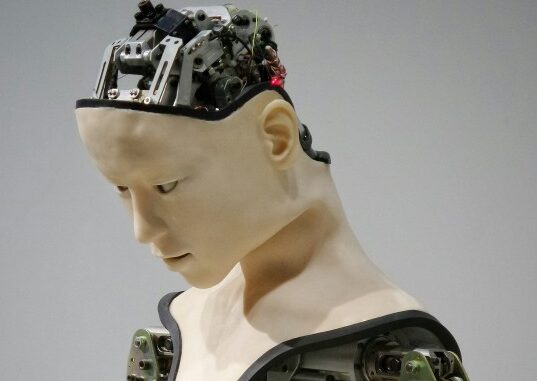
By Stuart Dee
The field of artificial intelligence (AI) is evolving rapidly, moving beyond the initial pursuit of ever-larger, all-encompassing language models toward a more nuanced and efficient approach: the development of smaller, highly specialised models coordinated by intelligent agents. This emerging paradigm promises to enhance accuracy, efficiency, and adaptability across various domains, presenting a transformative shift in how AI systems are designed and deployed.
The Case for Specialised Models
The growing preference for specialised models is rooted in their ability to deliver targeted, high-performance solutions. Unlike general-purpose large language models that require vast amounts of data and computational power, specialised models focus on specific tasks, enabling:
- Higher accuracy within their domain of expertise.
- Reduced data requirements for training.
- More frequent updates with domain-specific knowledge.
- Increased transparency and explainability.
- Lower environmental impact due to reduced computational demands.
These advantages make specialised models not only more efficient but also more adaptable to rapidly changing information landscapes.
The Power of Agentic AI Coordination
Central to the success of specialised AI ecosystems is the concept of agentic AI—autonomous systems that coordinate multiple specialised models, ensuring they work together seamlessly. These agents act as sophisticated orchestrators, dynamically managing model interactions and integrating outputs to produce cohesive results.
Key Functions of Agentic AI:
- Intelligent Routing and Coordination:
- Selects appropriate specialised models based on task requirements.
- Continuously assesses model performance.
- Optimises resource allocation.
- Context Management:
- Maintains contextual integrity across multi-model interactions.
- Manages memory and state in complex processes.
- Ensures consistency in long-running operations.
- Learning and Optimisation:
- Refines model coordination strategies.
- Learns from past successes and failures.
- Adjusts model weights and interactions for improved outcomes.
Financial Fraud Detection: A Case Study
Consider financial fraud detection—a domain where the integration of specialised models can significantly enhance accuracy and efficiency. In this context, agentic AI coordinates various models, each addressing specific aspects of fraud detection:
- Transaction Analysis Model:
- Monitors transaction patterns.
- Detects anomalies based on frequency, amount, and timing.
- Identifies known fraud behaviours.
- Customer Behaviour Model:
- Builds behavioural profiles from historical data.
- Detects deviations in individual customer habits.
- Document Verification Model:
- Authenticates financial documents.
- Identifies forged or altered paperwork.
- Geographic Risk Model:
- Evaluates regional fraud risks.
- Assesses cross-border transaction vulnerabilities.
The Agentic AI Coordinator orchestrates these models by:
- Assessing initial fraud indicators and selecting relevant models.
- Dynamically adjusting investigation paths based on findings.
- Synthesising insights to deliver a comprehensive fraud risk assessment.
Building the Framework: Technical and Ethical Considerations
Implementing agentic AI systems requires a robust framework that ensures seamless integration, security, and ethical compliance.
- Technical Integration:
- Orchestration Layers manage model interactions and conflict resolution.
- API Ecosystems standardise communication protocols and data formats.
- Meta-Models aggregate outputs and facilitate context-aware decision-making.
- Practical Challenges:
- Ensuring reliable decision-making and managing computational overhead.
- Maintaining system stability and handling complex edge cases.
- Ethical Considerations:
- Promoting transparency and accountability.
- Addressing bias and ensuring privacy protection.
Future Implications: Standardisation, Market Evolution, and Innovation
The shift towards specialised model ecosystems guided by agentic AI is likely to drive significant industry changes:
- Standardisation: Establishing common protocols and evaluation metrics.
- Market Evolution: Creating specialised model marketplaces and integration platforms.
- Technical Innovation: Advancing model coordination techniques and enhancing agent decision-making.
Conclusion
The future of AI lies not in the relentless scaling of single, massive models but in the intelligent integration of specialised models, each excelling in its niche. By leveraging agentic AI systems to coordinate these models, we can create smarter, more efficient networks capable of solving complex problems with precision and agility.
This approach not only mirrors human expertise—where specialists collaborate under effective leadership—but also paves the way for more sustainable, ethical, and adaptable AI solutions. As the AI landscape continues to evolve, success will hinge on mastering this balance between specialisation and coordination, unlocking new potentials across industries.