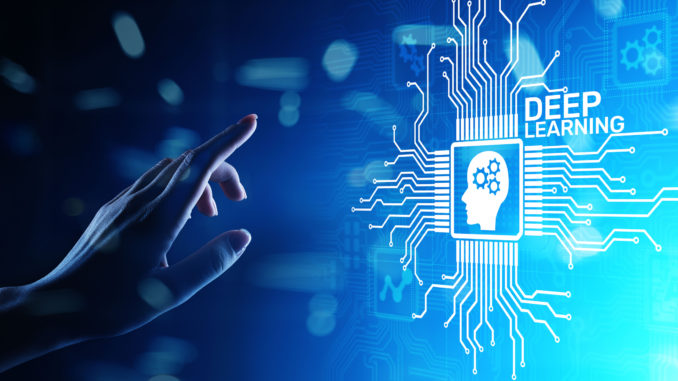
In Organizing Around Business Capabilities, my colleague Melissa Roberts and I made the case for structuring organizations around their business capabilities. We asserted that teaming structures organized around capabilities provide four significant sources of benefits, including stability, adaptability, accountability, and value maximization. Our article focused on organizing teams first to build, then to operate capability.
One of the topics we left unaddressed in our article was the impact that a capabilities-driven organization has on data strategy, architecture, and the AI-enabled business. Companies plan to spend $1 trillion on AI over the next 3 – 5 years, much of which will be wasted as they implement AI as a centralized, independent function rather than using AI to augment a capabilities-driven strategy.
A detailed exposition of the process from redesigning an organization structure to AI-enabled capabilities is beyond the scope of a single article, so for now I’d like to share a high-level point of view based on four key assertions.
- Organizing around capabilities enables domain driven data & analytics
- Organizing around capabilities aligns accountability for data quality with Capability Owners
- Items 1 and 2 enable a data mesh analytics architecture where data quality is managed at the source rather than the destination
- A data mesh architecture with clean source data enables vertical slicing / rapid deployment of AI-enhanced capability
Capabilities Inform Domain Decisions
Ever since Bill Inman coined the term “data warehouse” back in 1988, organizations have struggled to justify large, centralized cost structures that extract, transfer, clean and load data from operational systems into a format suitable for analytical processing. Centralized costs not only include the storage and compute costs for the analytics / data products, but also the overhead to establish centralized data governance and manage changes to schemas all along the data pipeline from operational system to warehouse.
More recent data architectures attempt to reduce these costs by changing the timing and location for data standardization and cleaning. The data lake architecture attempts to reduce processing costs relative to the warehouse architecture by deferring transformation until the data is needed for analysis, which allows more data to be initially stored at lower cost, but at the expense of additional preprocessing when it is used.
The data mesh architecture attempts to decentralize the data lake architecture by embracing domain driven design, aligning data ownership with business domains. This begs the question, “how do we identify the domains?” For organizations that are organized around business capabilities, the capability model is the obvious source for key business domains. Industry standard capability models such as the Business Architecture Guild’s BizBOK (subscription required) accelerate the domain discovery process because the capabilities themselves are organized around business objects such as Customer and Product.
Capability Owners Own Data Quality
As far back as my 2009 article, Business Capability Modeling: Theory and Practice, I have advocated that a business capability is an asset whose value should grow over time. If we accept that argument it is reasonable to include the cost of stewardship of the data in the cost to operate a capability. Including data stewardship in the operations of a capability aligns these costs with the value generated by the capability. The profitability requirements for a capability also act as a guardrail to bound the cost of data maintenance. Establishing accountability for data accuracy and cleanliness with a business capability solves a longstanding industry problem of centralized data maintenance costs that no single department wants to bear.
Enabling the Data Mesh
One of the advances of the data mesh architecture over the data lake architecture is that time to value is improved by decentralizing ownership of the data. Organizing around capabilities is a highly effective way to distribute responsibility for data management. Each capability team understands its data better than a centralized data organization. Also, decentralization increases the amount of work that can be done independently when there is sufficient coordination across capabilities to ensure consistency and interoperability.
One Slice at a Time
The clear connections between the business objects associated with a capability and business domains not only enable domain-driven services and applications, they make it possible to implement what Scott Ambler calls the “vertical slicing” approach to business intelligence. With this approach, an organization implements units of units of value from the data source to end user access in rapid timeframes. This approach is highly effective when value units are aligned with domains and the data at the source is clean. Vertical slicing is not only effective for business intelligence, but also relevant for the delivery of AI-enabled capability.
A Capabilities-Driven Approach to AI
Organizations often respond to trends in technology by developing centralized organizations to adopt the underlying technologies associated with a trend. The industry has decades of experience demonstrating that centralized approaches to adopting technology result in large, centralized cost pools that generate little business value. Since the past is often a good predictor of the future, we expect that many companies will attempt to adopt AI by creating centralized organizations or “centers of excellence,” only to burn millions of dollars without generating significant business value.
AI-enablement is much easier to accomplish within a capability than across an entire organization. Organizations can evaluate areas of weakness within a business capability, identify ways to either improve the customer experience and/or reduce the cost to serve, and target improvement levels. Once the improvement is quantified into an economic value, this value can be used to bound the build and operate cost of AI-enhanced capability.
Benefit and cost parameters are important because knowledge engineering is often the largest cost associated with an AI-enabled business process. Knowledge engineering includes collecting, manipulating, and analyzing the data that is embedded in models and used at runtime to guide a customer’s experience or make business decisions within a specific context.
When knowledge engineering costs are centralized through an “AI center of excellence,” they are sufficiently disconnected from the source of business value that the implementers wind up building solutions that are unprofitable to operate, and therefore are abandoned by the business units they are intended to help.
What’s Helpful to Centralize?
Centralization creates dependencies that must be orchestrated, and orchestration costs time and money. Therefore, we want to minimize the amount of work that is centralized to maximize the flow of value in capability development. That said, a completely decentralized model will be overcome by entropy that inhibits the interoperability of data assets.
I have observed this entropy in multiple organizations where, for example, customer information is managed in three or four different applications by different departments, none of which qualify as the single “source of truth” for the enterprise. Complicating matters further, the data attributes usually have different characteristics and semantics across the applications, making it very difficult to combine data from the source systems into a usable data product.
The ideal approach to governance is a federated governance model, where a small, centralized organization manages enterprise-wide concerns, collaborates with capability owners to ensure interoperability of data across domains, and provides access to specialized expertise needed to manage risk and security concerns. All other decision-making authority is delegated to individual Capability Owners who are then accountable to create and maintain high quality data within their capabilities, and to enable its interoperability with other capabilities or domains.
Standards & Interoperability
There is an entire industry and multiple professional associations devoted to data management, and hundreds if not thousands of books have been written on data governance. For our purposes, as organizations begin to deploy AI-enabled capabilities they need to establish a minimum viable set of standards for both AI and the underlying data that Robert Seiner describes as “non-invasive” [1]. That is, the governance mechanisms must be sufficiently “non-threatening” to make it easier to follow the governance than rebel against it. These mechanisms must explain not only how Capability Owners must describe (through metadata) and ensure sufficient data quality, but also how data product and AI-capability developers integrate operational data into new products and services.
A centralized organization must define at an enterprise level what is considered “critical data,” so Capability Owners prioritize quality of these data elements over others that are less critical. This organization must also establish decision-making authority within and across domains, so each major domain within the company has a known single source of truth, and the Capability Owner accepts responsibility for increasing the value of the data as an asset over time, with costs managed so that profitability of the capability increases as usage grows.
Security & Access Control
Next, the centralized governance must establish how stakeholders within and outside the organization obtain access to data and AI-enabled capabilities. These practices must be consistent across the organization, integrated with the organization’s identity management and role-based security mechanisms, and in an era of multi-tenant SaaS applications, provide ways for the organization’s customers to bring and manage their own keys and end user access to their data (and only their data). The security mechanisms must be sufficiently transparent to enable the organization to pass external audits.
Ethics
Another area that is important to centralize early in an organization’s journey to capabilities-driven AI is ethics. Why ethics? First, with the ease of access to cloud-based compute, storage, and machine learning algorithms, many teams have access to the tools needed to quickly produce a sophisticated AI model. Unfortunately, this makes it too easy for inexperienced people to unwittingly build models that are biased or unethical. Cathy O’Neil describes a situation like this in her book, Weapons of Math Destruction. She writes:
“From time to time, people ask me how to teach ethics to a class of data scientists. I usually being with a discussion of how to build an e-score [electronic scoring] model and ask them whether it makes sense to use ‘race’ as an input to the model. They inevitably respond that such a question would be unfair and probably illegal. The next question is whether to use ‘zip code.'” [2]
Since zip code in the United States is highly correlated with race / ethnicity, including it in a scoring model (credit worthiness, insurance risk, etc.) makes the model racially biased. Companies are increasingly being held accountable to prove to regulators that AI and machine learning algorithms used to make decisions are unbiased. Due to the “black box” nature of many of the machine learning algorithms, it is very difficult to prove a lack of bias after a model is built. Rather, “unbiased” must be intentionally designed into the process of developing a model. It won’t happen by accident. Centralized guidance is necessary to ensure that all teams building AI models comply with corporate policies as well as relevant laws wherever they do business.
A second important reason to establish enterprise-wide ethics policies is to guide the usage of information stored in knowledgebases of large language models (ChatGPT, Claude, Grok, etc.). One of the 2024 “game changers” in the AI space is the free or low-cost access that end uses now have to Generative AI tools. The Stanford 2024 AI Index Report [3] notes that OpenAI’s ChatGPT 4.0 used an estimated $78 million worth of compute to train its model. This $78 million resource is accessible to individual end users for $7 – 20 / month, depending on the service.
Low-cost access to Generative AI knowledgebases increases the urgency for companies to establish policies about their use of AI tools, such as:
- To the degree these models were created by extracting content from websites without compensation to the content copyright holders, is it ethical for your organization to include this content in its work products?
- Who within your organization makes this determination?
- How does it ensure the determination is consistently adopted across the organization?
- How does the organization cite source(s) for information accessed through a Generative AI tool?
Enterprise Business Value
Finally, and most importantly, a degree of centralized governance over data and AI capabilities to enable the company to quickly create new products and services across domains. The key role of centralized governance is to create the conditions that enable teams to rely on clean operational data and useful metadata consistently across domains. Governance ensures that individual domains are used correctly and retain their semantics / original meaning when integrated into a multi-domain AI model or data product.
Conclusions
Organizing around capabilities not only promotes effective and efficient business operations, but also streamlines capability development. In essence, it makes capabilities-driven AI possible. The capabilities-driven approach to AI accelerates delivery of data products and AI capabilities. As defined in the BizBoK, business capabilities are tied to business objects (or domains) with clear boundaries. These boundaries allow capability developers to independently work on a domain. Independence enables speed of delivery.
Independence at the domain level exposes the organization to the risk of inconsistent semantics across domains, which is an impediment to cross-domain AI capabilities. To mitigate this risk, a small amount of centralized governance is necessary to ensure interoperability and consistency of data semantics, as well as security, enterprise business value, and ethical use of data and algorithms.
Len has over 30 years of experience helping large organizations generate billions of dollars in economic value by leading high risk, high visibility business and digital transformations. He currently serves as Chief Scientist at LeadingAgile, the leader in helping companies grow in value through business agility. He leads engagements with large clients to help them improve flow by restructuring value delivery around their business capabilities, consistently delivering 30 – 85% improvements in productivity, quality, and business value. Len received Bachelor and Master of Arts degrees in Sociology from the University of Illinois at Chicago with concentrations in Organization Theory, Research Methods and Statistics. He can be reached at leonard.greski@leadingagile.com.
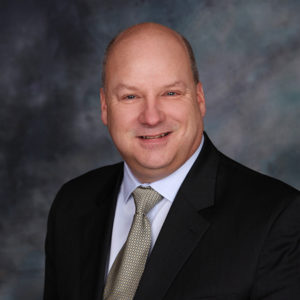
References
[1] Seiner, Robert – Non-Invasive Data Governance Strikes Again: Gaining Experience and Perspective, Technics Publications, Sedona AZ, 2023 p. 10.
[2] O’Neil, Cathy – Weapons of Math Destruction: how big data increases inequality and threatens democracy, Crown, New York, NY, 2016 pp. 145 – 146
[3] Perault, Ray and Jack Clark (Eds.) – Artificial Intelligence Index Report 2024, AI Index Steering Committee, Institute for Human-Centered AI, Stanford University, Stanford CA, 2024, p. 5.