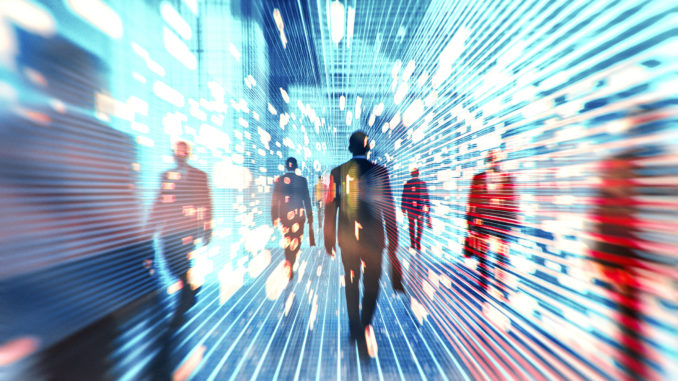
Big data has disrupted industries in waves, compounding the technology adoption process complexity. Disruptions from digital transformation has accelerated obsolescence of existing enterprise data management architectures and increased the pressure on IT teams to migrate to big data platforms. Despite the heavy investment in time and resources to establish the big data- enabled architecture, businesses have not seen value from this transformation. Below we address three reasons why the business value and adoption from big data implementation is still lagging.
Technology complexity
The first reason is related to the complexity of an evolving technology that has delayed achieving a “production ready” platform. Technology teams have had to work through several iterations managing to the new waves of technology changes.
The first wave addressed the “volume” of big data. Enterprise technology teams worked on solving the data integration, storage and migration challenges presented by the volume of digital data, by introducing distributed systems, like the data lake on-prem or on cloud. Legacy platforms built on traditional appliance servers like Oracle, Teradata, or IBM mainframes were still being retained for ongoing operations. The next wave addressed the “variety” of digital data. Greater focus was placed on integrating collection and storage of unstructured data like call- center recordings, social media feeds, images, granular click stream and chat logs, for downstream users. Before the technology team tackled processes to handle the “velocity” of new data creation or the third wave, implementation progression was tied in knots creating protocols and processes to manage data security, governance, and encryption to address the fourth challenge of big data – “data veracity”. Lost in these efforts were user accessibility and relevant use case testing milestones.
Imbalance between Big data adoption strategies of technology and analytic teams
Balancing technology adoption with usability goals in a holistic manner was overlooked as resources were focused on meeting the technology transformation milestones. Due to the potential delays in platform implementations, business teams set out on an independent strategy to work with big data. This disconnect with the technology growth plan further delayed business adoption of the new platform.
Business organization models have traditionally placed the charge of technology and innovation with the CIO team, while the CMO and the strategy teams have led the charge for creating and delivering revenue. The CIO team, as cost centers, are focused singularly on technology adoption. CMO teams, as revenue centers, have had to independently design and modify business practices to deliver value of big data insights to the leadership teams with existing or acquired tools and capabilities. This has led to misalignment in the technology adopted within an enterprise – even if it was initially allowed as a temporary solution.
Marketing departments adopt new tools or techniques to develop and maintain a competitive edge in the markets. The use of SEO, clickstream analytics, customer journey analytics, next recommended solutions, sentiment analysis, digital/ online marketing are ways that marketing has responded to leveraging the variety and volume of big data available to them. Working with analytic teams, digital data expertise has been developed using existing/ legacy architecture and databases. As an intermediate milestone, technology team provided expanded analytic capabilities on existing platforms as the new platform was either “under development” or inaccessible to the business teams. With growing demand and availability of big data analytic tools, the business teams turned to external vendors and vendor applications to enable delivery against business goals.
The delays in accessibility to the platform for business users led to a widening gap as teams adopted alternate tools and technology to reach their goals (see figure below). The value-add being delivered by the business team was not associated with the enterprise technology transformation but the use of tools and features outside the enterprise big data architecture.
Getting back on plan not “build and they will come”
The third reason delaying the adoption, once the technology transformation has been completed, has been the difficulty in closing the gap resulting from the independent execution of big data transformation. The extended timelines and the instability of the platform technology has challenged the transition of business to the new platform and created resistance to migrate to an untested environment.
The CIO department has made substantial investments in time and budget to handle the big data disruption based on a business model of delivering “art of the possible” in 5 or 10 years. This planned architecture did not anticipate the rapidity of the technology disruptions. A stable masterplan for a big data framework is no longer realistic. This has been the Achilles heel for the technology team that has delayed accessibility till stability and secure governance processes were successfully established.
For the business team extended timelines, lack of familiarity, and low confidence levels in the performance of the platforms have created resistance to change and transition. The resistance can be overcome if, at early build stages, several of the elements were considered and implemented by the leadership teams. A small list of factors that are contributing to the delays in business adoption are listed below.
- Lack of successful customization and end-user satisfaction tests through business relevant use cases / pilots on the new integrated platform
- Lack of availability of a stable environment or analytic features/ tools matching those currently used to deliver against business goals
- Lack of bridge teams to communicate and socialize the transition process effectively
- Lack of clarity around roles, accountability, and performance across the technology and business teams
- Lack of an informed and collaborative analytic culture.
These factors are holding back and delaying the accelerated adoption of the big data platform. Enterprises with Big Data projects are addressing the first couple of factors listed above. The last 5 are associated with qualitative and “soft” assets which require a new perspective. Future articles will provide details on the build up of an analytic culture and the layers of “soft” architecture.