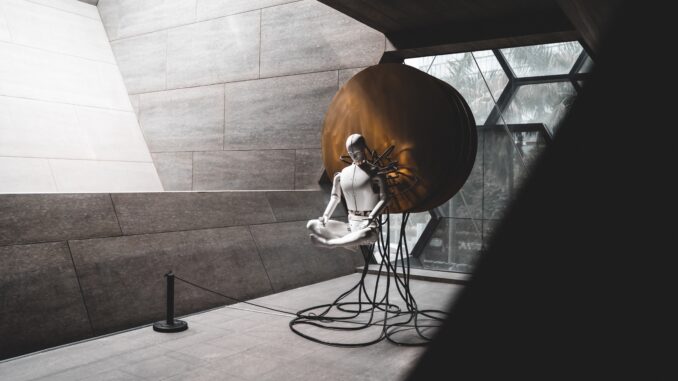
By Maria Vaida, Ph.D., Assistant Professor of Data Science at Harrisburg University of Science and Technology
We are currently witnessing a significant transformation in data-engineering processes driven by AI-powered tools – particularly in automation, predictive analytics, and real-time decision-making. These advancements are set to significantly boost productivity and creativity, and expand access to technology for non-technical users across just about every industry on earth.
Data pipelines – the backbone of modern data management – consist of systems and processes that move data through stages of extraction, transformation, and loading (ETL) to prepare it for analysis. They play an essential role in every industry that relies on data-driven insights, acting as conduits that enable organizations to process massive amounts of data and generate valuable information.
AI-driven tools are revolutionizing these data pipelines – from collection and cleansing to storage and analysis – while requiring minimal human intervention. By utilizing large language models (LLMs) and advanced machine learning algorithms, we can now automate complex workflows and develop adaptive pipelines that seamlessly adjust to new data structures. For instance, retrieval-augmented generation (RAG) and fine-tuning pipelines improve LLMs by automatically integrating, cleaning, and organizing data, while simultaneously detecting and resolving quality issues in real-time, all while safeguarding data privacy. This greatly reduces the need for manual coding, minimizes errors, enhances accuracy, and ensures that data remain secure and ready for analysis.
With recent advancements in artificial intelligence (AI), data pipelines are transforming at an unprecedented rate. They’re becoming faster, more flexible, and more accessible, even to non-technical users. As AI-powered tools revolutionize data pipelines, entire industries are witnessing substantial impacts to their productivity, creativity, and decision-making capabilities.
Automating Data Engineering for Efficiency and Innovation
AI’s role in the data pipeline begins with automation, especially in handling and processing raw data – a traditionally labor-intensive task. AI can automate workflows and allow data pipelines to adapt to new data formats with minimal human intervention. With this in mind, Harrisburg University is actively exploring AI-driven tools for data integration that leverage LLMs and machine learning models to enhance and optimize ETL processes, including web scraping, data cleaning, augmentation, code generation, mapping, and error handling. These adaptive pipelines, which automatically adjust to new data structures, allow companies to manage large and evolving datasets without the need for extensive manual coding.
One AI approach revolutionizing data handling is retrieval-augmented generation (RAG), where LLMs access external data sources to generate accurate, real-time insights. This technique enables pipelines to incorporate data automatically, detect errors, and resolve inconsistencies as they arise, ensuring data quality without requiring manual cleansing. These processes not only reduce the time required to prepare data but also enhance its reliability. For instance, Google Cloud’s AutoML Tables can analyze and transform data structures, helping businesses reduce ETL labor costs considerably and allowing companies to focus on gleaning insights rather than getting bogged down in preparation.
AI-Driven Predictive Analytics in Industry
Predictive analytics has emerged as another powerful AI tool transforming data pipelines. Traditional analytics rely on correlation analyses, but AI can uncover causal relationships within datasets, opening new possibilities for industries like finance and entertainment.
In financial services, predictive analytics helps institutions anticipate market fluctuations and manage risk. By analyzing real-time data from multiple sources, including social media, economic indicators, and past market performance, AI-driven pipelines can identify early signals of economic downturns or potential market opportunities. A study by Deloitte found that companies implementing AI-powered predictive models saw up to a 20% improvement in risk mitigation strategies. These predictive capabilities help businesses adjust their investment strategies, maintain liquidity, and ensure regulatory compliance.
At Harrisburg University, our research also explores how LLMs can go beyond traditional correlation analysis to uncover causality in datasets, opening new possibilities for industries such as entertainment. For example, we are studying how AI can analyze movie scripts and audience feedback to predict a film’s success. By pinpointing trends in character arcs, plot structures, and viewer reactions, AI can help filmmakers make more informed decisions about story elements that resonate with audiences, potentially increasing profitability in what is already a multi-billion-dollar industry.
In healthcare, our research integrates AI models with real-time blood test results and medical literature – including publications and biomedical databases – to enable early detection of diseases like cancer and Alzheimer’s disease. This capability accelerates diagnoses and allows for timely, potentially life-saving interventions. Such innovations in data-driven diagnostics could save millions in treatment costs and reduce mortality rates. Similarly, we are examining how businesses can harness AI-driven tools, such as LLM-powered systems, to analyze real-time financial and customer data from both structured and unstructured sources, helping them make better decisions and improve risk management.
In the business sector, real-time decision-making enables companies to adapt quickly to market changes. With AI-driven tools analyzing both structured and unstructured financial and customer data, businesses can monitor consumer preferences, track competitor activities, and predict customer churn. Amazon.com uses an AI-based pipeline that leverages real-time sales data to optimize its inventory and distribution networks, reducing holding costs while improving delivery times. This real-time adaptability makes businesses more agile, responsive, and competitive.
Addressing Ethical Challenges in AI-Driven Pipelines
Despite these advancements, this transformation also poses challenges. Our research addresses critical ethical issues surrounding AI and LLMs, including hidden biases in the training datasets, the potential long-term impact of LLMs on low-resource languages, and the need to ensure that AI technologies are applied responsibly, benefiting society while mitigating potential risks.
Hidden biases within AI training datasets can affect the accuracy and fairness of data outputs, leading to unintended consequences. Bias in data pipelines, for instance, has impacted industries such as banking, where biased lending algorithms inadvertently disadvantage certain demographic groups. Additional research is required to create bias-mitigation techniques and ensure that AI-driven tools are trained on diverse datasets, in order to minimize bias and promote inclusivity.
Moreover, the application of LLMs in low-resource languages presents another ethical challenge. AI models trained predominantly in high-resource languages may not perform well in languages with limited digital content, limiting access to AI technology for speakers of these languages. This disparity can perpetuate existing inequalities in access to technology and digital services. By encouraging multilingual dataset creation, researchers can extend the benefits of AI-driven data pipelines to a wider audience, promoting equity in technological advancements.
AI for Scalable and Sustainable Data Solutions
Beyond immediate operational improvements, AI is shaping the future of scalable and sustainable data pipelines. As industries collect data at an accelerating rate, traditional pipelines often struggle to keep pace. AI’s ability to scale data handling across various formats and volumes makes it ideal for supporting industries with massive data needs, such as retail, logistics, and telecommunications.
In logistics, for example, AI-driven pipelines streamline inventory management and optimize route planning based on real-time traffic data. FedEx’s AI-powered logistics platform has reduced delivery times and cut fuel consumption, reducing environmental impact and lowering operational costs by million of dollars annually.
The Future of AI-Driven Data Pipelines
The future of data pipelines lies in continuous learning and autonomous adaptation, which AI facilitates. Adaptive pipelines that learn from historical data and refine their processes over time could soon become the norm. Additionally, the convergence of AI with edge computing and IoT (Internet of Things) devices allows pipelines to process data closer to its source, reducing latency and improving real-time decision-making. In manufacturing, IoT-enabled data pipelines can detect equipment malfunctions as they occur or even beforehand, which reduces downtime and associated expenses.
Although additional ethics-focused research is required, this is an incredibly fruitful area of study already. By integrating AI into data pipelines, industries can unlock new levels of productivity, creativity, and adaptability – ultimately driving economic growth and innovation.