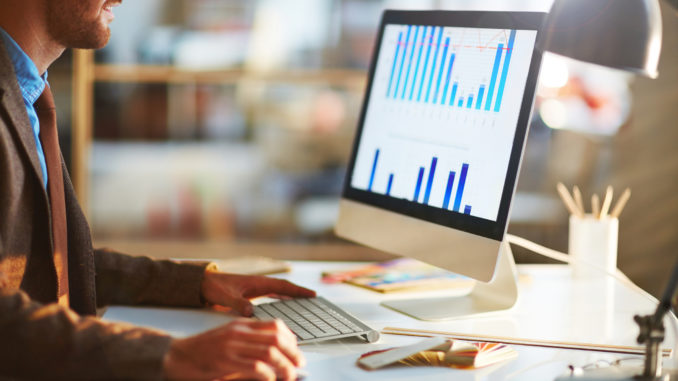
Today, Generative AI (GenAI) technology has become so disruptive and has changed the way enterprises do business.
Return on Investment (ROI) provides a thorough understanding of how to calculate and assess the value delivered by GenAI initiatives. Measuring the Return on Investment (ROI) of Generative AI adoption is crucial for enterprises to justify their investments and ensure they are achieving the desired outcomes.
ROI of the GenAI initiatives depends on the following factors,
- Driving Innovation
- Enhancing Productivity
- Creating new business opportunities
- Quantifying the benefits of GenAI
- Evaluation of financial metrics
- Strategic impact of AI investments
This article introduces the fundamental concepts of ROI, structured framework of ROI, essential steps to calculate ROI, explores various ROI metrics, and discusses the advantages and challenges of using an ROI-based approach in GenAI adoption. Article acts like a guide to businesses in effectively calculating the potential ROI for projects, ensuring they make informed decisions before implementation.
Industry Trends
According to Gartner, “Any organization in any industry, especially those with very large amounts of data, can use AI for business value.” Generative AI is primed to make an increasingly strong impact on enterprises over the next five years.
- Gartner Predicted that, “In 2026, 75% of CDAOs that have failed to make organization-wide influence their top priority will have been assimilated back into technology functions.”
- According to IDC global study of 2023, for every $1 companies invest in AI, they are realizing an average of $3.5 in return and 5% of organizations worldwide are realizing an average of $8 in return.
- According to Microsoft survey, Organizations using AI experienced an 18% increase in Customer satisfaction, Employee productivity and market share.
- Generative AI is rated to be the top emerging enterprise technology. 80% believe that it will disrupt their industry and nearly all (93%) think generative AI will provide value to their business – KPMG
- Generative AI coding support can help software engineers develop code 35 to 45 percent faster, refactor code 20 to 30 percent faster, and perform code documentation 45 to 50 percent faster-McKinsey
- By 2027, nearly 15% of new applications will be automatically generated by AI without a human in the loop. This is not happening at all today – Gartner
- Recent CEO surveys show almost 80% of CEOs believe AI is likely to significantly enhance business efficiencies in their organization – Forbes
- Today, an estimated 60% of IT leaders are looking to implement Generative AI – CIO.com
Approach to Calculate ROI
ROI is not a number. Measuring ROI of GenAI is a complex process. It’s about optimizing investment and ensure GenAI delivers a strong return for business.
Calculating the ROI (Return on Investment) for Generative AI (GenAI) adoption involves the steps that covers cost savings, revenue generation and total cost of ownership. The steps involved are,
- Define Objectives
- Review GenAI Initiatives
- Measure Key Metrics
- Calculate the Cost
- Estimate Benefits
- Calculate ROI
- Monitoring ROI
The following section briefs the activities to performed in each step and the role of IT in brief.
Define Objectives
Define a clear vision and mission for GenAI implementation aligned with the enterprise goals and business Strategy. An effective AI vision of an enterprise is forward-looking and aspirational and reflects the enterprise’s commitment to leveraging AI to deliver positive and responsible outcomes.
The Objective is to harness the power of GenAI technologies to support and drive enterprise innovation, efficiency, business growth, and resilience. The goal is to establish the metrics that addresses,
- Reduced operational costs
- Increased business sales
- Higher customer satisfaction
- Faster time to market for new products
Review GenAI Initiatives
Review existing technological infrastructure, data availability, skill pool, and existing AI capabilities. Identify high impact GenAI use cases based on potential impact and strategic alignment. Define enterprise GenAI Roadmap that guides to deliver the GenAI applications. GenAI usage processes help to monitor and audit the GenAI systems.
Measure Key Metrics
To realize the benefits of the GenAI Investments, it’s critical to establish Key metrics early in the process of AI implementation. These metrics need to match business goals and objectives.
Metrics help to ensure that the enterprise is staying on track with respective to implementation of GenAI initiatives. It ensures that the cost of implementation and actual results are on par with expectations.
GenAI team needs to continuously refine and improve how to use AI, as well as how to measure success across enterprise. Most of the key Metrics include cost savings, revenue growth, Productivity gains, customer satisfaction, time saved, Marketing Effectiveness and operational efficiency.
Calculate the Cost
The cost covers environment setup cost, tool setup and its usage cost, training cost, cost of governance, use case implementation cost and ongoing cost.
- Environment Cost: covers setting up of development environment, Setting up of Test, QA and Production Environment
- Tools Usage Cost: It includes AI Initiatives that get derived from the tools. For ex: SAP Success Factor, Service Now, Dynamic O365, Power BI
- Training Cost: It covers Developer Training, Business Training, Tool Training and Leadership training. Investing in training programs to enhance the GenAI capabilities of cross-functional teams, ensuring they remain up to date with the latest AI trends and technologies.
- Advisory Services Cost: It covers 3rd Party Advisory Services, current GenAI Maturity Assessment.
- Governance: It includes a Centrally Managed Budget (Technology Cost, Consulting cost) and BU Managed Budget (HR, Legal etc). Adoption of AI Tools, Monitoring. Establish tools, technologies, and platforms to support AI governance Monitoring involves, controlling model performance, detect data drift, retraining models
- Cost for High Impact Use cases: Based on strategic alignment and potential impact on the business the identification of the Use cases and the resourcing cost for implementing them need to be estimated.
- Ongoing Costs: covers maintenance, updates, and any additional staffing requirements.
Estimate Benefits
The following parameters help to estimate the benefits of Generative AI adoption,
- Cost savings from automation, increased sales from improved customer interactions, or reduced time to market.
- Identify new customer journeys and identify new audiences. Improve customer interactions through enhanced chat and search experiences
- Improvements in employee productivity, better decision-making, and enhanced innovation capabilities. Transform campaigns, audiences, experiences, journeys and insights.
- Help marketing teams think of better ideas, execute campaigns faster and create more highly personalized experiences and reach the right customers
Calculate ROI
To calculate the ROI of GenAI across the enterprise, we need to consider the cost of the implementation and the expected benefits. Estimate the cost savings, increase in revenue and other benefits that AI can bring to the enterprise.
(Revenue increase from GenAI – Cost of AI Implementation)
ROI = ———————————————————————— × 100%
(Cost of AI Implementation)
If enterprise GenAI project costs $400,000 and generates $600,000 in benefits (cost savings and additional revenue), then ROI would be:
(600,000−400,000)
ROI = ˗————————× 100% = 50%
400,000
Monitoring ROI
Continuously track the performance of GenAI initiatives and adjust enterprise strategy as needed to maximize ROI.
Challenges in Measuring ROI
The main challenges faced by the enterprises today in measuring ROI Generative AI solutions are,
Data requirements: Identification of data sources for AI, labeling of data for algorithms, data management, data governance, data policies, data security, and data store are the challenges for the enterprises. Poor data quality significantly affects the performance of GenAI models. Ensuring relevant data is crucial for accurate ROI calculations.
Reliability: Trained models are black boxes and has no clue to end user. This may lead to false, harmful and unsafe results. This may lead to rework which incurs additional cost and time affecting ROI.
Technology complexity: Data preparation for LLMs, algorithm design, building of models, training the models is a complex task. Compute identification for training, cloud identification and deployment are complex tasks. Realizing this complexity can lead to inaccurate cost and time estimates.
Huge Customization: Enterprise business needs require extensive Fine tuning of base foundation models and prompt engineering.
Skill Gap: Generative AI initiatives require investments in Machine Learning/Deep Learning/Prompt Engineering/Large Language Model expertise to build and train Foundation Models to run the enterprise systems. Many enterprises lack these skilled resources and are not available in-house. Enterprises building algorithms and models to meet the business requirement will be a challenge.
Lack of Governance: AI models degrade over time leading to reduced accuracy and effectiveness. Inability to rapidly respond to AI technology changes driven by business/technology challenges and no holistic enterprise AI view. Data becomes stale or obsolete and hence becomes useless or less useful impairing strategic decision-making based on this stale/obsolete data thereby defeating the very purpose of AI.
Expectations of Enterprise: Unrealistic expectations about the capabilities and timelines of GenAI initiatives leads to rework and higher cost.
Ongoing Cost: GenAI projects require continuous monitoring, maintenance and updates
Siloed GenAI Projects: Implementing GenAI initiatives in Siloed way leads to mismatch of the enterprise business strategy, higher cost and no benefits.
Best Practices for Adoption of Generative AI
The following are the GenAI best practices that transforming the industry,
- Establish culture of responsible AI: Build use cases and minimum viable products, focus on governance, guardrails, prototype delivery systems, change management, and prioritization of use cases.
- Incorporate auditing: Perform Auditing that helps businesses develop and deploy policies to protect the business from risks such as copyright infringement and proprietary data leakages.
- Create Center of Excellence: Center of excellence upskill employees in generative AI, they can learn to adjust the prompts AI uses in the initial stages and fine tune in the later stages to address inaccurate and biased outputs.
- Democratize Ideas, Limit Production: Prevent employees from launching untested and unregulated AI projects.
- Prepare for dynamic data: Vast amount of dynamic data that GenAI creates include tables, code, and images. Enterprise leaders must work with agility to streamline data sources, talent, and technology.
- Bring in the Business: With GenAI, business executives to be intrigued, ambitious and vocal about what they could achieve with AI.
Conclusion
The use of Generative AI across enterprises is becoming more and more widespread, possibly even trending toward industrialization.
GenAI can deliver significant ROI by increasing efficiency, enhancing decision-making, improving customer experiences, and driving revenue growth.
Understand Generative AI fundamentals to identify business use cases. The enterprise GenAI Strategy guide in aligning AI initiatives to the enterprise business drivers. It needs to be aligned with enterprise business strategy. GenAI costs vary depending on several factors, including the initial assessment, project scope and complexity, customization and integration requirements, and ongoing support and maintenance.
Calculate the ROI by estimating the potential cost savings, revenue increase, and other intangible benefits that GenAI can bring to the enterprise. Compare these benefits to implementation costs to determine the overall value.
Acknowledgements
The author would like to thank Santosh Shinde of BTIS, Enterprise Architecture division of HCL Technologies Ltd for giving the required time and support in many ways in bringing this article as part of Architecture Practice efforts.
About Author
Dr. Gopala Krishna Behara is a Enterprise Architect in BTIS Enterprise Architecture division of HCL Technologies Ltd. He has a total of 28 years of IT experience. Reached at gopalakrishna.behara@hcl.com.
Disclaimer
The views expressed in this article/presentation are that of authors and HCL does not subscribe to the substance, veracity or truthfulness of the said opinion.