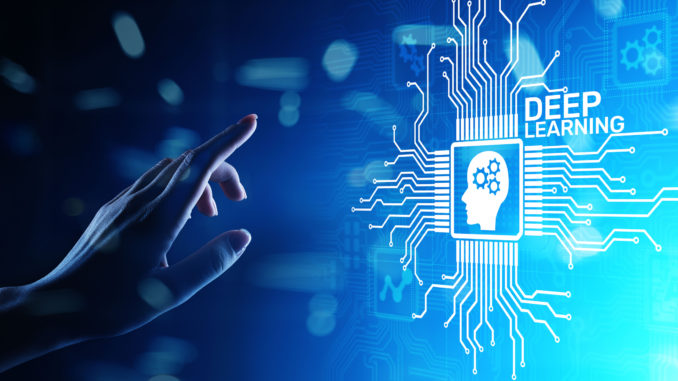
By Jitendra Gupta, Head of AI & Data Science, Wolters Kluwer ELM Solutions
This summer, chipmaker Nvidia became the most valuable public company, surpassing Microsoft. In the past year alone, its market cap tripled to $3 trillion, driven primarily by the artificial intelligence (AI) boom. Tech giant Apple also announced it is entering the generative AI race, solidifying the technology’s staying power. Indeed, both traditional and generative AI boast truly revolutionary potential. Nearly 80% of corporate strategists think AI will be critical to their success in the years ahead, per Gartner, while the AI industry writ large is expected to grow by almost 30% each year through 2030.
For many companies, the question is not whether they should implement AI, but how and when. Some organizations are opting to build their own models in-house—an approach that can be extremely resource-intensive, though beneficial from a security perspective. According to Gartner, though, less than half of AI projects actually make it into production, and the path from prototype to production can take months, if not years. Put another way, it’s an undertaking that should only commence after tremendous consideration.
When deciding whether to buy or build an AI solution, companies must assess three things: data, resources, and available partners. Let’s take a closer look at each.
- Data
Any AI solution is only as smart as the data it’s trained on. Data is AI-ready when it’s complete, clean, readable, and free of bias. Organizations should ask themselves if they have a good grasp of available data and its quality before embarking on an AI journey of any kind. Ideally, the organization will have access to high-quality internal data and external sources, such as industry benchmark data. Without sufficient, high-quality data, building an in-house model isn’t even an option.
If access to external data is lacking, choosing an AI solution that contains the requisite data can be a good choice. Still, you may need to feed your company’s data into a large language model (LLM)—something that must be done with extreme care. If company data is being sent out to the public domain, it can undermine privacy and security—particularly for industries, like the legal industry, that deal with sensitive information.
The ideal approach is for an AI vendor to use retrieval-augmented generation, or RAG, which allows the model to be optimized using a knowledge base beyond what it was trained on. But internal data should only be fed to the model with guardrails in place. All internal data should be kept within a delineated sandbox and not shared with external sources unless it is completely anonymized and approved by the customer.
- Resources
In addition to quality data, organizations must also assess whether they have the resources—both talent and money—to create and manage an AI solution long-term. Training an AI model is not cheap; ChatGPT cost $10 million to train in its current form, while the cost to develop the next generation of AI systems is expected to be closer to $1 billion. Traditional AI tends to cost less than generative AI because it runs on fewer GPUs, yet even the smallest scale of AI projects can quickly reach a $100,000 price tag.
Building an AI model should only be done if it’s expected that you will recoup building costs within a reasonable time horizon. Additionally, building requires a company to have data science expertise for ongoing support and maintenance. Models must be continually analyzed, tested and updated to ensure accuracy, while data, as already mentioned, must be diligently maintained. When buying an AI model, on the other hand, vendors often provide the data scientists required of maintenance.
- Partners
Because building an AI model is so time- and resource-intensive, organizations should ask themselves if they have a trusted partner with an AI model they can use instead. The right partner will help integrate new AI applications into the existing IT environment and, as mentioned, provide the talent required for maintenance. Choosing an existing model tends to be cheaper and faster than building a new one. Still, the partner or vendor must be vetted carefully.
Vendors with an established history of developing AI will likely have better data governance frameworks in place. Ask them about policies and practices directly to see how transparent they are. Are they flexible enough to make said policies align with yours? Will they demonstrate proof of their compliance with your organization’s policies? The right partner will be prepared to offer data encryption, firewalls, and hosting facilities to ensure regulatory requirements are met, and to protect company data as if it were their own. With these assurances, partnering with a vendor that uses AI allows organizations to reap the benefits of the technology without having to integrate and maintain AI in their environments.
The bottom line is that AI is here to stay—but reaping its benefits can be difficult. Many organizations will likely be tempted to dive in by building their own model in-house, but they must make sure they have the requisite data, talent, and time for such an undertaking. It’s often far more efficient and cost-effective to buy an existing model and safely supplement it with company data—but only if the vendor has top-notch security and a track record of success.