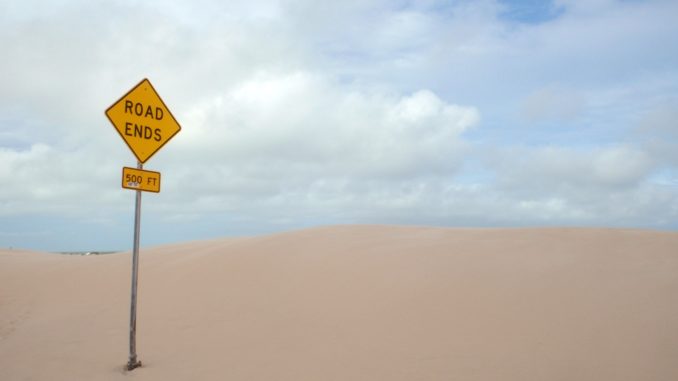
It is more likely than not, that data is a huge, barely tapped resource in your organisation. Anecdotal evidence suggests that traditional organisations have mostly recognised that data is a key asset and using the insight gained from a company’s data is more important than ever. In practice, newer organisations, disruptors and start-ups have been much more dynamic and agile in exploiting data to shake-up, compete, and win in your markets. And, if they are using that data well, then they will be cherry picking your prime customers. It is quite likely they may not have as much data as you, but they are more able to exploit it.
Big Data is the buzz word that has defined a series of approaches that many companies have used to develop the solutions that create the insight needed. However, statistics from Gartner and others show that the majority of companies have failed to realise their goals using a Big Data approach.
The core of a Big Data approach is to centralise the ingestion, transformation, governance and insight across a large portion of an organisations data. This leads to bottlenecks that significantly inhibit the delivery of business value in meaningful timeframes. Rather than enabling the flow of data it has become strangled.
Is there an alternative?
What if there was a new approach based on federation rather than centralisation to help businesses gain the insight they need to remain competitive?
We have been working with a number of organisations and have found for many of them the Data Mesh approach addresses the challenges they are facing. At its core, Data Mesh is a federated approach to data based on tried and tested principles of software engineering many organisations already apply to customer journey development.
The Three Principles of Data Mesh
The Data Mesh approach leverages three core principles from modern software engineering: –
- domain ownership,
- product development, and a
- self-service software platform.
These principles enable federation of the development of data solutions which in turn can unlock significant greater insight more quickly so organisations can realise business value.
Domain Ownership
This takes current domain modelling principles and puts a data overlay on the model. A domain model is a visual representation of the key concepts/objects of a problem domain, at the highest level for the whole of an organisation. It decomposes an organisation to establish clear ownership and boundaries for both business capabilities and technology solutions. This enables a microservices based approach to software engineering that drives autonomy and reuse.
This same domain model can be used within an organisation to establish ownership of data sets for a domain. Each data set should be owned by the domain that creates/generates the data. The aim is to have each data set owned by a single domain, tweaking the domain model where needed to achieve this outcome.
Product Development
This suggests that an organisation treat data as a product in the same way as they treat customer journeys as products.
The focus is on the customer, the jobs they want to do and the best solution to help them do this. The product team is a multi-skilled set of individuals that bring business and technology people together to create the best customer outcome possible.
Applying this to data, means understanding the different data personas across an organisation which include customers, internal business users, other engineering teams, B2B partners and regulators. This will help define the jobs to be done for the different user groups allowing the product team to focus on solving those challenges for each group of users. Product development aligned with domain ownership will create clear lines of accountability for data sets and user outcomes enabling teams to move at pace.
Self-service Software Platform
A self-service software platform for data is at the heart of enabling the autonomy and agility needed to deliver of the outcomes of the Data Mesh approach. At its core, an organisation needs to think like a cloud service provider and create an API driven, self-service data platform. This platform needs to provide three sets of capabilities starting with base infrastructure for storage, databases, access control, etc. Engineering tools for workflow to abstract away the complexities of the infrastructure via Infrastructure as code and DevOps. Finally, the platform needs to provide central management capabilities of discovery, compliance and monitoring.
Implementing the three principles of Data Mesh helps remove the bottlenecks inherent in the Big Data approach. With the data platform in place, each product team can define their backlog of data work to do and prioritise the different user outcomes based on value release to the organisation. Each product team can work with autonomy, at speed, within the investment budget they have been allocated.
How to set up for success?
Implementing the Data Mesh approach requires domain modelling and product development as part of the normal software engineering lifecycle. Successfully implementing domain ownership and product development impacts people, skills and organisational design which has significant ramifications and need buy in from senior stakeholders to be implemented successfully.
Many companies are already transforming their organisations in this way and including a Data Mesh approach as part of the wider transformation should reduce the overall effort and cost involved in implementing Data Mesh. Where an organisation has changed their operating model, the Data Mesh approach is the logical next step to drive further value from the model and alleviate many of the challenges inherent in the Big Data approach.
The other critical factor in successfully implementing Data Mesh, is to ensure the self-service data platform is set up correctly. This requires some up-front thinking to define the capabilities, architecture and team skills and structures the platform needs to enable autonomy for the product teams. It also requires a team who understands the vision for the self-service platform and has the skills needed to implement it.
Finally, we recommend starting small. Create an MVP self-service platform enabling a small set of non-critical data sets to prove both the technology and operating model in the context of your organisation.
Silver bullet?
Data Mesh can overcome many of the challenges inherent in the Big Data approach by driving greater levels of autonomy and federation of data engineering across a wider set of stakeholders. However, it is not a silver bullet and introduces a different set of risks for an organisation to manage.
With an understanding of the risks that come with a Data Mesh approach and a plan on how to mitigate these risks, the right architecture to underpin the self-service platform and stakeholders that support the vision, a step change in an organisations ability to leverage data can be made.
Principal Architect at Enterprise Blueprints since 2010, Neil Mulholland has been involved with Digital and Integration architecture since the 1990s. Lately he’s been instrumental in helping move organisations to Agile methods at scale with a focus on how architecture and architectural governance can work within this environment.
Neil believes trust is earned through a demonstrated ability to focus on what matters most for the client. Understanding the client’s needs, and achieving the outcomes they want, is essential for defining successful solutions and building long-term, mutually-beneficial partnerships.
Neil contributes to his clients’ organisation through informal and formal mentoring of their architecture teams, helping upskill their entire architecture team with better solutions and operational methods. He’s a great fan of experimenting with new technology and learning how best this can be applied to solve customer problems, but dislikes steep learning curves when introducing new technology that can delay real adoption. His expertise has seen him work for IBM, Telstra, National Australia Bank, Barclays Global Investors, Lloyds Banking Group, Travelex, CSC and DWP.